Algorithms
ab-initio prediction of protein structure: An introduction
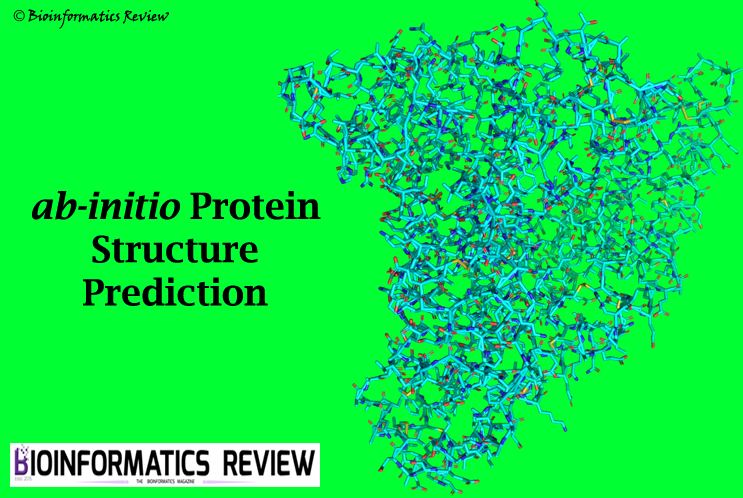
We have heard a lot about the ab-initio term in Bioinformatics, which could be difficult to understand for newbies in the field of bioinformatics. Today, we will discuss in detail what ab-initio is and what are the applicable methods for it.
First of all, let’s get familiar with the literal meaning of the term ab-initio, it means ‘from the scratch’. This term is applied in the context of the protein structure prediction in bioinformatics, which is quite useful. Actually, ab-initio is one of the methods to predict a protein structure, which in case not available in protein data bank (PDB) [1]. There are basically three methods to predict a protein’s structure:
a) homology modeling
b) ab-initio
c) threading
Homology modeling method is applied when there is a sufficient amount of similarity between the protein (structure to be predicted) and the template (whose structure have been determined already). But in the other case, when the similarity between the two is quite low, then the ab-initio method is applied. Although homology modeling aims to find a template protein which is evolutionary related to the query protein sequence. Threading is a little similar to homology modeling in the sense that it predicts the structure by recognizing the folds of the template and it aims to detect the evolutionary-related proteins and analogous folds, so we can say they are template-based methods. The homology modeling and threading both are capable of predicting protein structures with high-resolution folds based on the searched templates, but they suffer a few limitations that the native topology for the query sequence must have been solved, and new folds cannot be predicted using these two approaches.
The ab-initio method is often preferred for structure prediction when there is no or very low amount of similarity for the protein (let’s say query protein sequence). It is the most difficult [2,3] and general approach where the query protein is folded with a random conformation. The ab-initio method is based on the thermodynamic hypothesis proposed by Anfinsen [4], according to which the native structure corresponds to the global free energy minimum under a given set of conditions.
There are several ab-initio structure prediction approaches available such as ROSETTA [5], TOUCHSTONE-II [6], and the most widely preferred I-Tasser [7,8]. These approaches are based on the Monte-Carlo algorithm [9,10]. It has been found that I-Tasser outperforms the ROSETTA and TOUCHSTONE-II approaches with a far lower CPU cost [11].
The ab-initio modeling is often termed as de-novo modeling [12], physics-based modeling [13], or free modeling [14]. The basic protocol followed by the ab-initio method of the protein structure prediction starts with the primary amino acid sequence which is searched for the different conformations leading to the prediction of native folds. After the folds have been recognized and predicted, the model assessment is performed to verify the quality of the predicted structure. ROSETTA and I-Tasser follow the enhanced methodology for ab-initio prediction of a protein.
ROSETTA prediction begins with the identification of small fragments (3mers and 9 mers) from the structure databases that have consistency with local sequence preferences. After that, all the fragments are assembled into models with global properties followed by the assessment of the models using a scoring function from decoy population [5]. The protocol followed by the I-Tasser includes threading along with the ab-initio method [6,7]. I-Tasser program is based on the secondary-structure enhanced Profile-Profile threading Alignment (PPA) [15] and the iterative implementation of the Threading ASSEmbly Refinement (TASSER) program [16]. The details of the I-Tasser program can be read here.
We will be discussing other protein structure methods in detail in the upcoming articles.
References
- Protein data bank (www.rcsb.org)
- Lu, L., Lu, H., & Skolnick, J. (2002). MULTIPROSPECTOR: an algorithm for the prediction of protein-protein interactions by multimeric threading. Proteins: Structure, Function, and Bioinformatics, 49(3), 350-364.
- Floudas, C. A., Fung, H. K., McAllister, S. R., Mönnigmann, M., & Rajgaria, R. (2006). Advances in protein structure prediction and de novo protein design: A review. Chemical Engineering Science, 61(3), 966-988.
- Anfinsen, C. B. (1973). Principles that govern the folding of protein chains. Science, 181(4096), 223-230.
- Simons, K. T., Bonneau, R., Ruczinski, I., & Baker, D. (1999). Ab initio protein structure prediction of CASP III targets using ROSETTA. Proteins: Structure, Function, and Bioinformatics, 37(S3), 171-176.
- Zhang, Y., Kolinski, A., & Skolnick, J. (2003). TOUCHSTONE II: a new approach to ab initio protein structure prediction. Biophysical journal, 85(2), 1145-1164.
- Wu, S., Skolnick, J., & Zhang, Y. (2007). Ab initio modeling of small proteins by iterative TASSER simulations. BMC biology, 5(1), 17.
- Zhang, Y. (2008). Progress and challenges in protein structure prediction. Current opinion in structural biology, 18(3), 342-348.
- Simons, K. T., Kooperberg, C., Huang, E., & Baker, D. (1997). Assembly of protein tertiary structures from fragments with similar local sequences using simulated annealing and Bayesian scoring functions. Journal of molecular biology, 268(1), 209-225.
- Hansmann, U. H., & Okamoto, Y. (1999). New Monte Carlo algorithms for protein folding. Current opinion in structural biology, 9(2), 177-183.
- Wu, S., Skolnick, J., & Zhang, Y. (2007). Ab initio modeling of small proteins by iterative TASSER simulations. BMC biology, 5(1), 17.
- Bradley, P., Misura, K. M., & Baker, D. (2005). Toward high-resolution de novo structure prediction for small proteins. Science, 309(5742), 1868-1871.
- Ołdziej, S., Czaplewski, C., Liwo, A., Chinchio, M., Nanias, M., Vila, J. A., … & Schafroth, H. D. (2005). Physics-based protein-structure prediction using a hierarchical protocol based on the UNRES force field: assessment in two blind tests. Proceedings of the National Academy of Sciences of the United States of America, 102(21), 7547-7552.
- Jauch, R., Yeo, H. C., Kolatkar, P. R., & Clarke, N. D. (2007). Assessment of CASP7 structure predictions for template free targets. Proteins: Structure, Function, and Bioinformatics, 69(S8), 57-67.
- Wu, S., & Zhang, Y. (2007). LOMETS: a local meta-threading-server for protein structure prediction. Nucleic acids research, 35(10), 3375-3382.
- Zhang, Y., & Skolnick, J. (2004). Automated structure prediction of weakly homologous proteins on a genomic scale. Proceedings of the National Academy of Sciences of the United States of America, 101(20), 7594-7599.
Algorithms
MOCCA- A New Suite to Model cis- regulatory Elements for Motif Occurrence Combinatorics
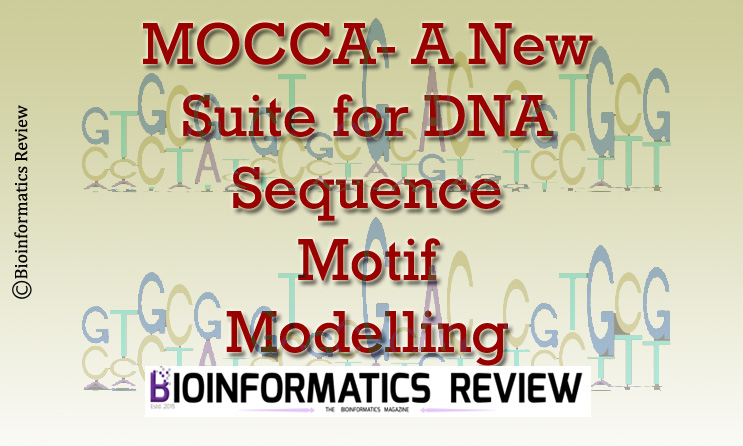
cis-regulatory elements are DNA sequence segments that regulate gene expression. cis-regulatory elements consist of some regions such as promoters, enhancers, and so on. These regions consist of specific sequence motifs. (more…)
Algorithms
vs_Analysis.py: A Python Script to Analyze Virtual Screening Results of Autodock Vina
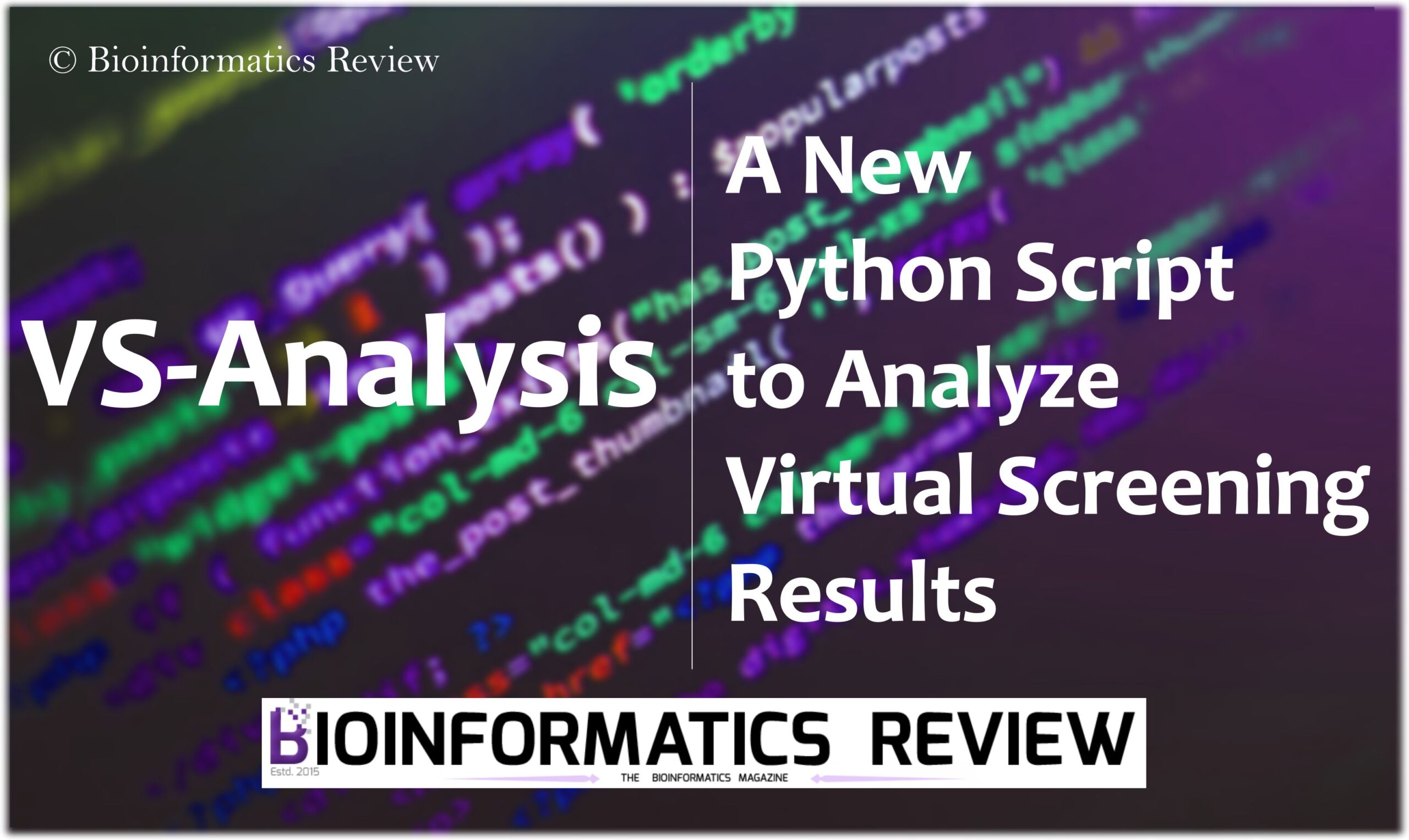
The output files obtained as a result of virtual screening (VS) using Autodock Vina may be large in number. It is difficult or quite impossible to analyze them manually. Therefore, we are providing a Python script to fetch top results (i.e., compounds showing low binding affinities). (more…)
Algorithms
How to search motif pattern in FASTA sequences using Perl hash?
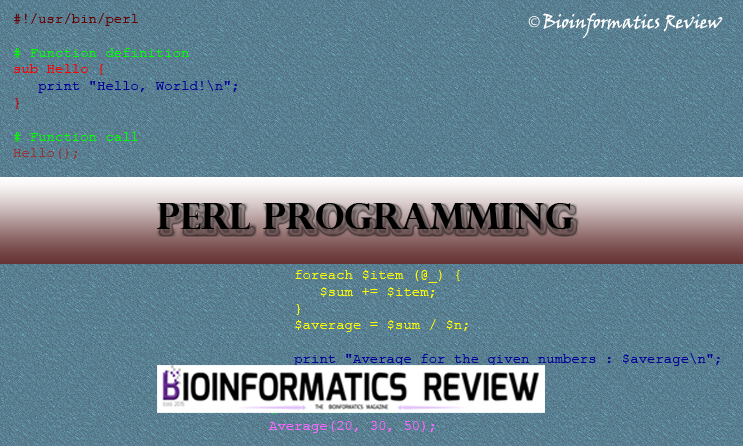
Here is a simple Perl script to search for motif patterns in a large FASTA file with multiple sequences.
Algorithms
How to read fasta sequences from a file using PHP?
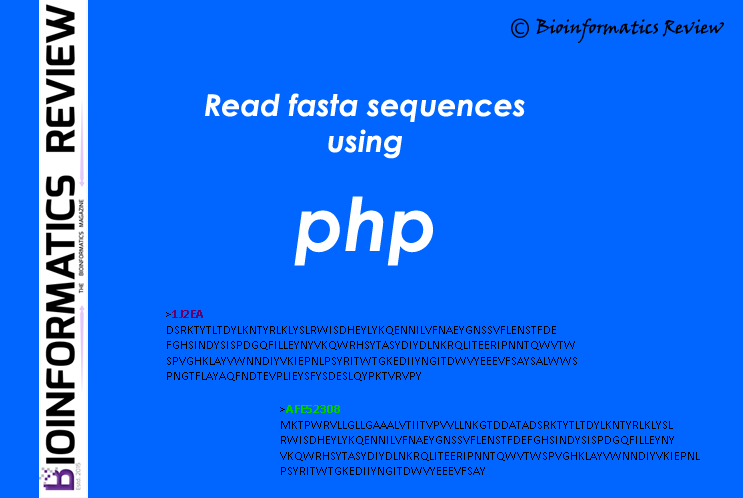
Here is a simple function in PHP to read fasta sequences from a file. (more…)
Algorithms
How to read fasta sequences as hash using perl?
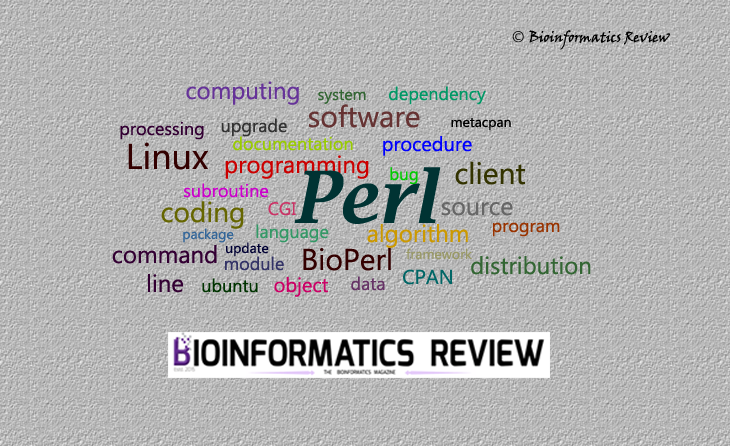
This is a simple Perl script to read a multifasta file as a hash. (more…)
Algorithms
BETSY: A new backward-chaining expert system for automated development of pipelines in Bioinformatics
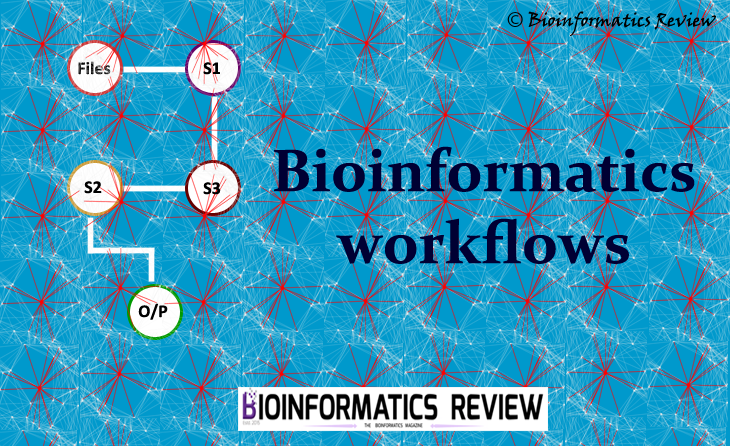
Bioinformatics analyses have become long and difficult as it involves a large number of steps implemented for data processing. Bioinformatics pipelines are developed to make this process easier, which on one hand automate a specific analysis, while on the other hand, are still limited for investigative analyses requiring changes to the parameters used in the process. (more…)
Algorithms
Algorithm and workflow of miRDB
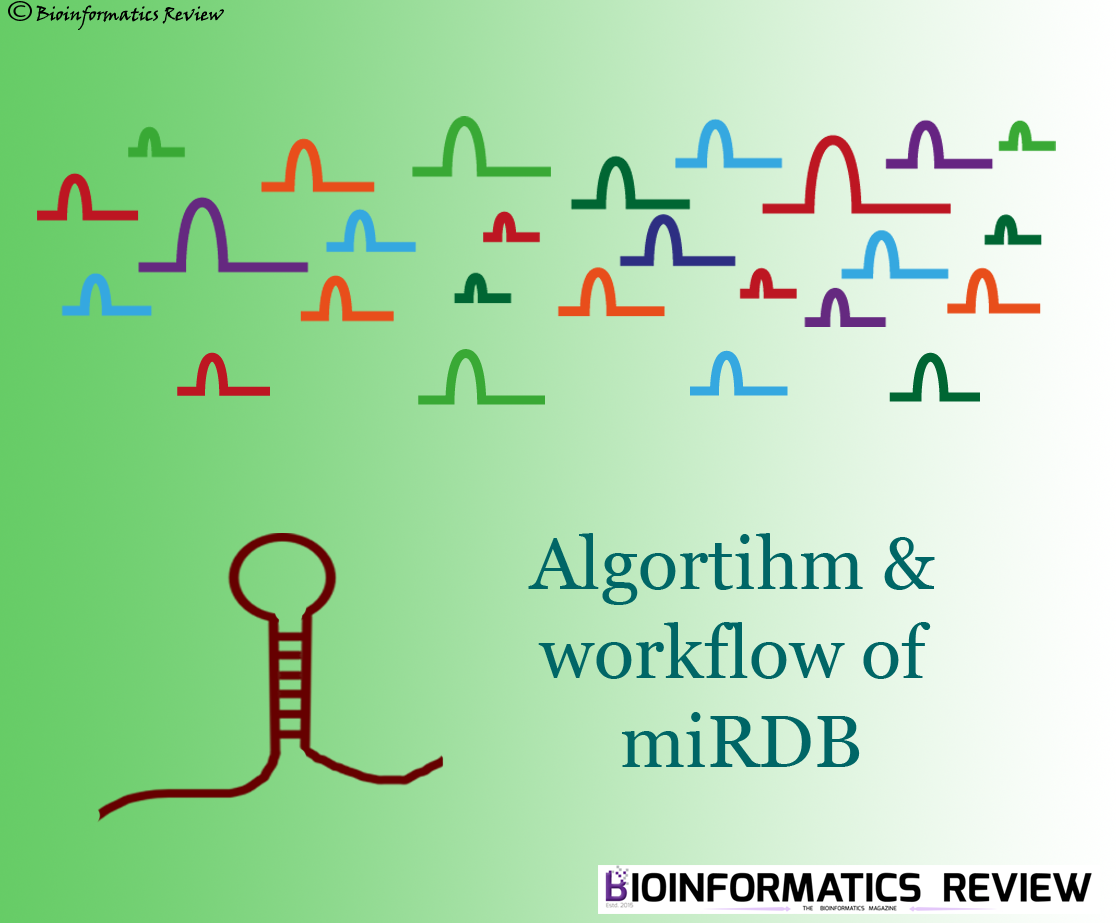
As mentioned in the previous article, Micro RNAs (miRNAs) are the short endogenous RNAs (~22 nucleotides) and originate from the non-coding RNAs [1], produced in single-celled eukaryotes, viruses, plants, and animals [2]. They play significant roles in various biological processes such as degradation of mRNA [3]. Several databases exist storing a large amount of information about miRNAs, one of such databases miRBase [4] was explained in the previous article, today we will explain the algorithm of miRDB [5,6], another database for miRNA target prediction. (more…)
Algorithms
miRBase: Explained
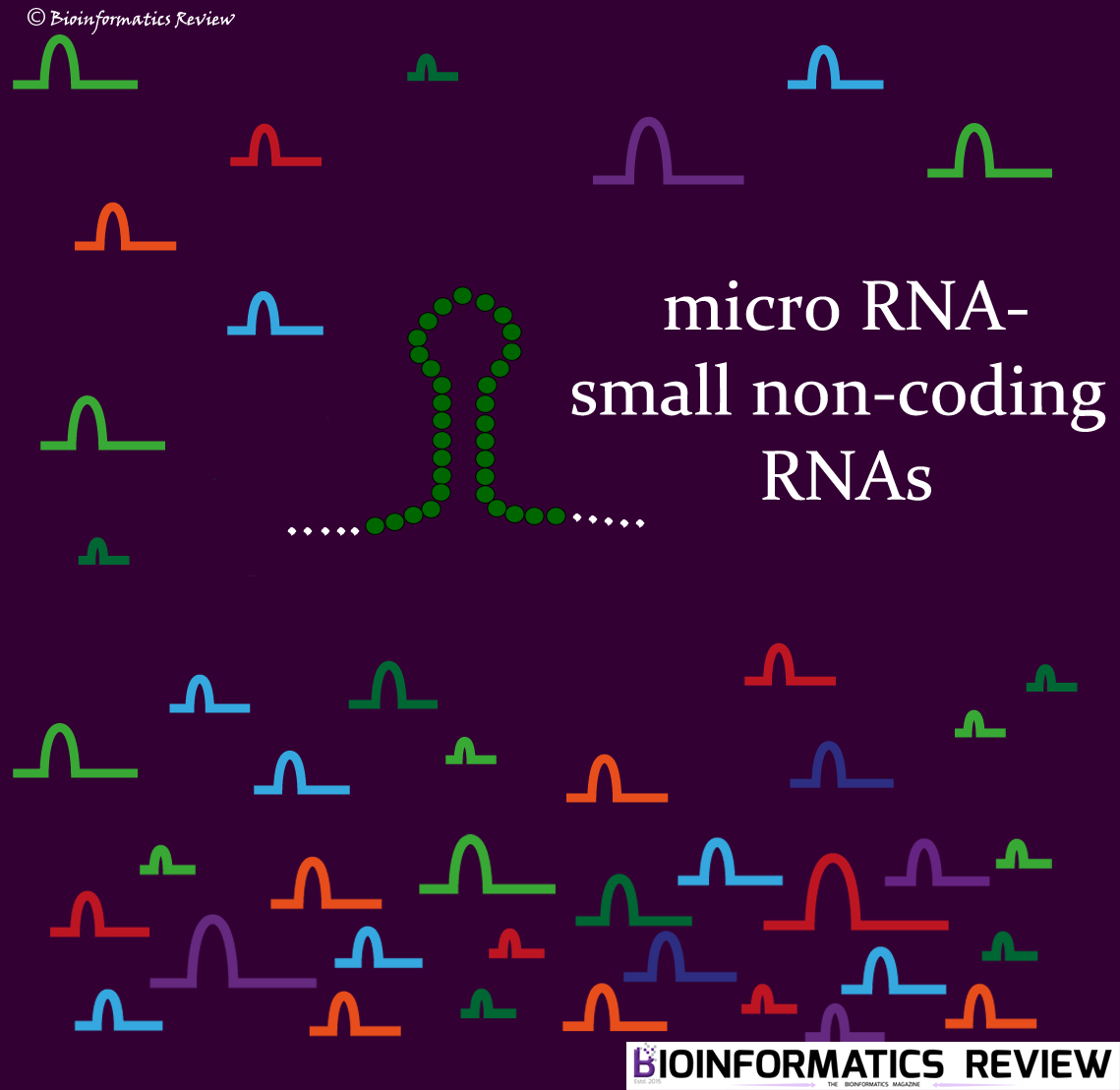
Micro RNAs (miRNAs) are the short endogenous RNAs (~22 nucleotides) and originate from the non-coding RNAs [1], produced in single-celled eukaryotes, viruses, plants, and animals [2]. miRNAs are capable of controlling homeostasis [2] and play significant roles in various biological processes such as degradation of mRNA and post-translational inhibition through complementary base pairing [3]. (more…)
Algorithms
Prediction of biochemical reactions catalyzed by enzymes in humans
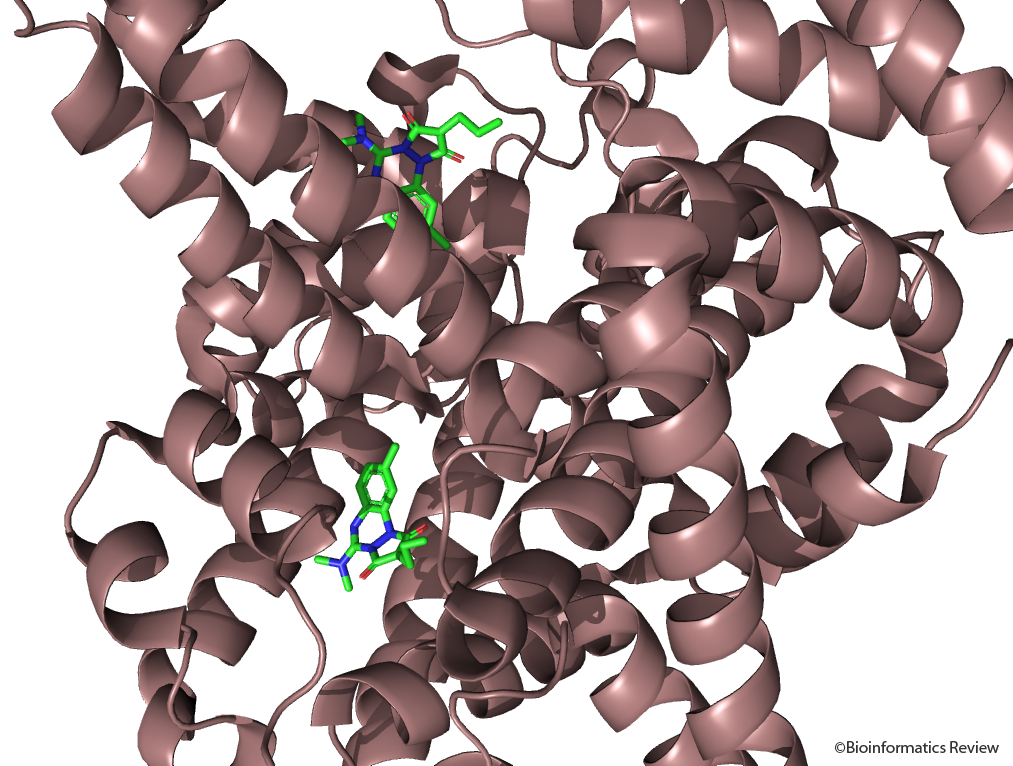
There are many biological important enzymes which exist in the human body, one of them is Cytochrome P450 (CyP450) enzymes which are mostly considered in drug discovery due to their involvement in the majority (75%) of drug metabolism [1]. Therefore, various in-silico methods have been applied to predict the possible substrates of CyP 450 enzymes [2-4]. Recently, an in-silico model has been developed to predict the potential chemical reactions mediated by the enzymes present in humans including CyP450 enzymes [5]. (more…)
Algorithms
A new high-level Python interface for MD simulation using GROMACS
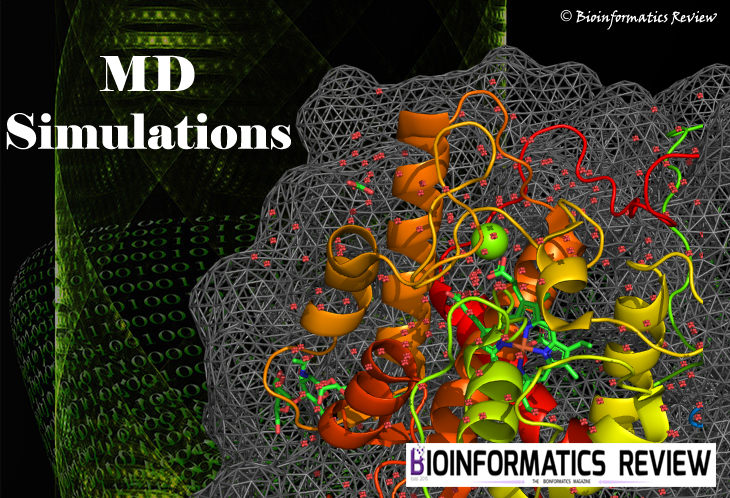
The roots of the molecular simulation application can be traced back to physics where it was applied to simplified hard-sphere systems [1]. This field of molecular simulation study has gained a lot of interest since then and applied to perform simulations to fold small protein at multi-microsecond scale [2-4], predict functional properties of receptors and to capture the intermediate transitions of the complex [5], and to study the movement and behavior of ligand in a binding pocket and also to predict interactions between receptors and ligands [6,7]. (more…)
Algorithms
Machine learning in prediction of ageing-related genes/proteins
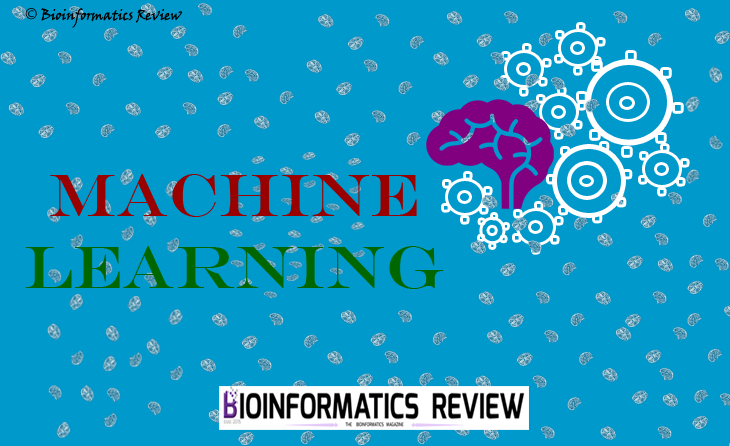
Ageing has a great impact on human health, when people’s age advance towards 80 years, approximately half of the proteins in the body get damaged through oxidation. The chemical degradations occurring in our body produce energy by the consumed food via oxidation in the presence of oxygen. (more…)
Algorithms
Simulated sequence alignment software: An alternative to MSA benchmarks
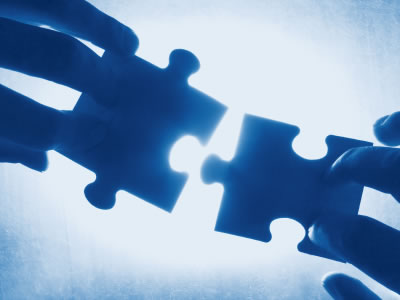
In our previous article, we discussed different multiple sequence alignment (MSA) benchmarks to compare and assess the available MSA programs. However, since the last decade, several sequence simulation software have been introduced and are gaining more interest. In this article, we will be discussing various sequence simulating software being used as alternatives to MSA benchmarks. (more…)
Algorithms
Benchmark databases for multiple sequence alignment: An overview
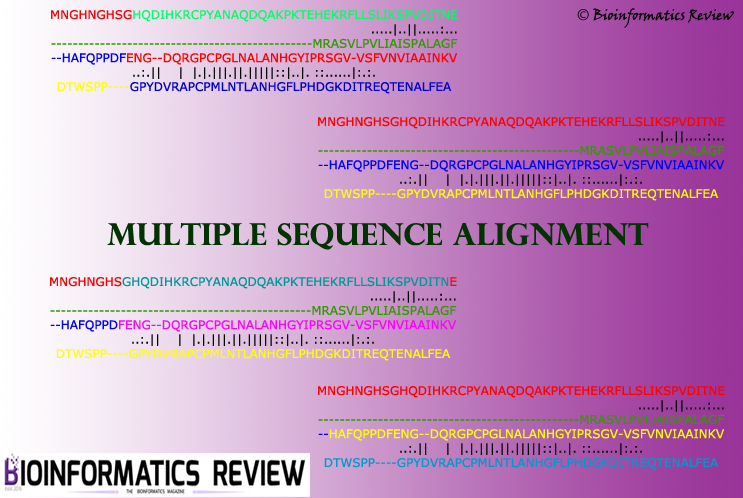
Multiple sequence alignment (MSA) is a very crucial step in most of the molecular analyses and evolutionary studies. Many MSA programs have been developed so far based on different approaches which attempt to provide optimal alignment with high accuracy. Basic algorithms employed to develop MSA programs include progressive algorithm [1], iterative-based [2], and consistency-based algorithm [3]. Some of the programs incorporate several other methods into the process of creating an optimal alignment such as M-COFFEE [4] and PCMA [5]. (more…)
Algorithms
Intrinsically disordered proteins’ predictors and databases: An overview
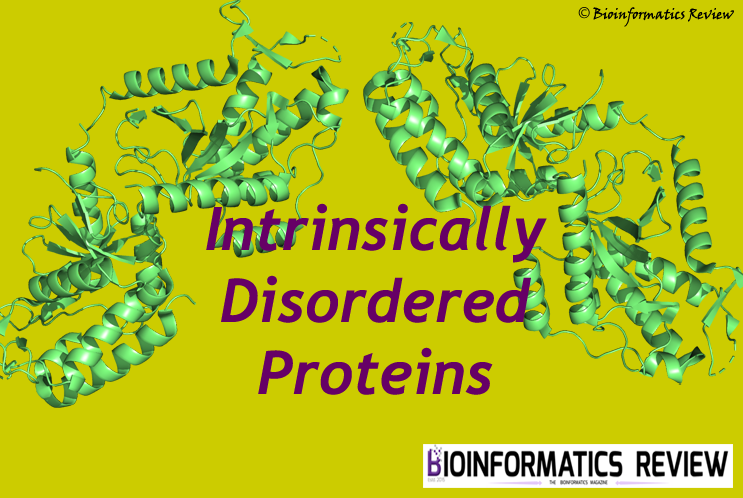
Intrinsically unstructured proteins (IUPs) are the natively unfolded proteins which must be unfolded or disordered in order to perform their functions. They are commonly referred to as intrinsically disordered proteins (IDPs) and play significant roles in regulating and signaling biological networks [1]. IDPs are also involved in the assembly of signaling complexes and in the dynamic self-assembly of membrane-less nuclear and cytoplasmic organelles [1]. The disordered regions in a protein can be highly conserved among the species in respect of both the composition and the sequence [2]. (more…)
Algorithms
An introduction to the predictors of pathogenic point mutations
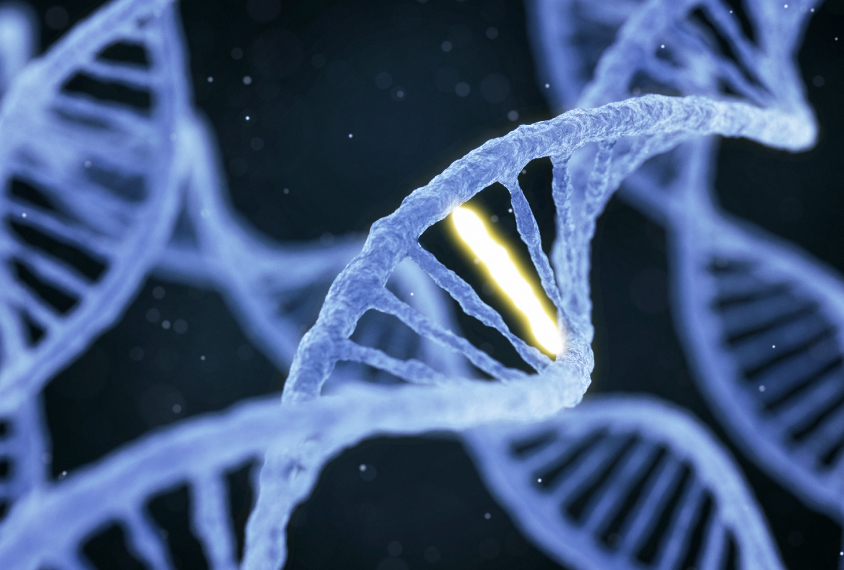
Single nucleotide variation is a change in a single nucleotide in a sequence irrespective of the frequency of the variation. Single nucleotide variants (SNVs) play a very important role in causing several diseases such as the tumor, cancer, etc. Many efforts have been made to identify the SNVs which were initially based on identifying non-synonymous mutations in coding regions of the genomes. (more…)
Algorithms
SparkBLAST: Introduction
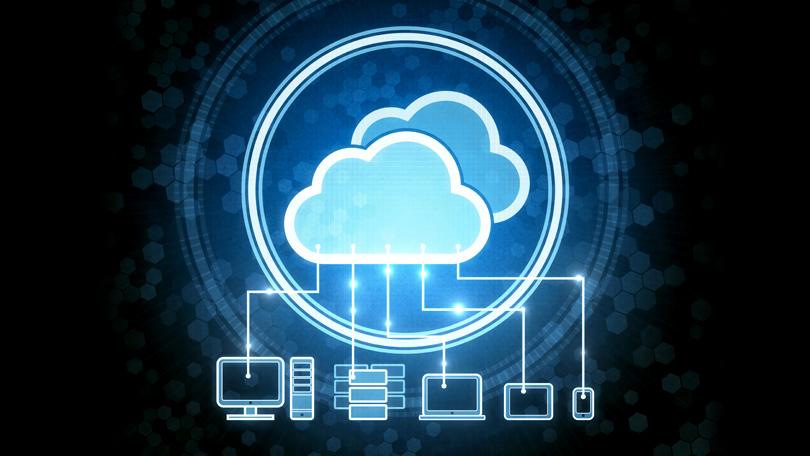
The basic local alignment search tool (BLAST) [1,2] is known for its speed and results, which is also a primary step in sequence analysis. The ever-increasing demand for processing huge amount of genomic data has led to the development of new scalable and highly efficient computational tools/algorithms. For example, MapReduce is the most widely accepted framework which supports design patterns representing general reusable solutions to some problems including biological assembly [3] and is highly efficient to handle large datasets running over hundreds to thousands of processing nodes [4]. But the implementation frameworks of MapReduce (such as Hadoop) limits its capability to process smaller data. (more…)
Algorithms
Role of Information Theory, Chaos Theory, and Linear Algebra and Statistics in the development of alignment-free sequence analysis
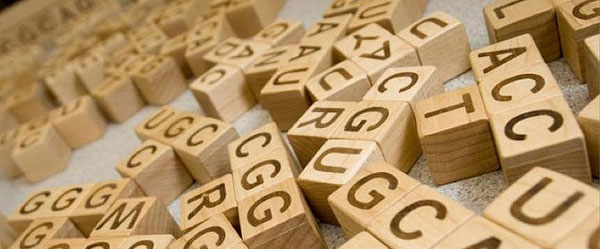
Sequence alignment is customary to not only find similar regions among a pair of sequences but also to study the structural, functional and evolutionary relationship between organisms. Many tools have been discovered to achieve the goal of alignment of a pair of sequences, separately for nucleotide sequence and amino acid sequence, BLOSSUM & PAM [1] are a few to name. (more…)
Algorithms
Bioinformatics Challenges and Advances in RNA interference
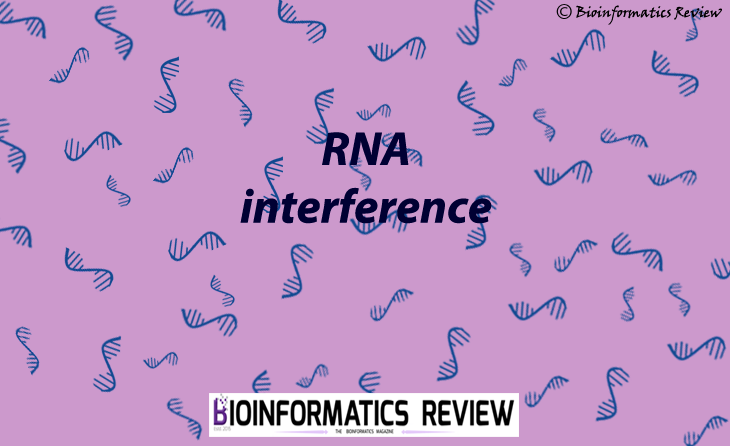
RNA interference is a post-transcriptional gene regulatory mechanism to down-regulate the gene expression either by mRNA degradation or by mRNA translation inhibition. The mechanism involves a small partially complementary RNA against the target gene. To perform the action, it also requires a class of dedicated proteins to process these primary RNAs into mature microRNAs. The guide sequence determines the specificity of the miRNA. Therefore, the knowledge of the guide sequence is crucial for predicting its targets and also exploiting the sequence to create a new regulatory circuit. In this short review, we will briefly discuss the role and challenges in miRNA research for unveiling the target prediction by bioinformatics and to foster our understanding and applications of RNA interference. (more…)
Algorithms
Systems pharmacology and drug development
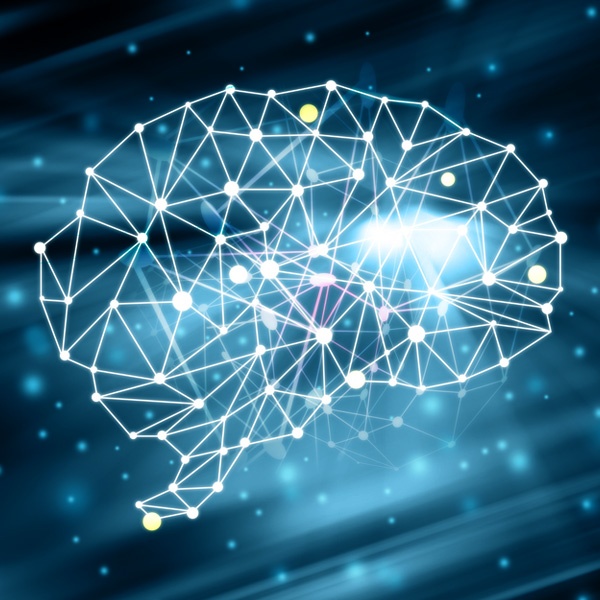
Systems pharmacology is an emerging area in the field of medicinal chemistry and pharmacology which utilizes systems network to understand drug action at the organ and organism level. It applies the computational and experimental systems biology approaches to pharmacology, which includes network analyzes at multiple biological organization levels facilitating the understanding of both therapeutic and adverse effects of the drugs. Nearly a decade ago, the term systems pharmacology was used to define the drug action in a specific organ system such as reproductive pharmacology [1], but to date, it has been expanded to different organ and organism levels [2]. (more…)
Algorithms
Recent advances in in-silico approaches for enzyme engineering
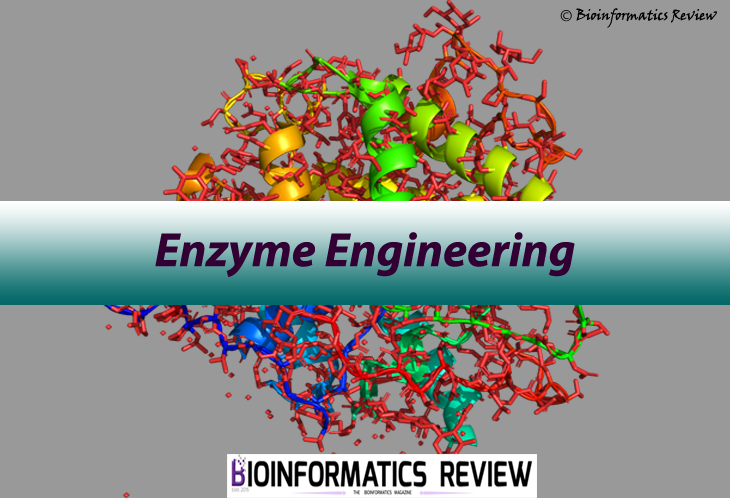
Enzymes are natural biocatalysts and an attractive alternative to chemicals providing improved efficiency for biochemical reactions. They are widely utilized in industrial biotechnology and biocatalysis to introduce new functionalities and enhance the production of enzymes. In order to be proved beneficial for industrial purposes, the enzymes need to be optimized by applying protein engineering. This article specifically reviews the recent advancements in the computational approaches for enzyme engineering and structural determination of the enzymes developed in recent years to improve the efficiency of enzymes, and the creation of novel functionalities to obtain
products with high added value for industrial applications. (more…)
You must be logged in to post a comment Login