In a network, integration of elements and interacting components enables identification of conserved modules and
motifs. The topological analysis, however, reveals much about the nature and functions of a network and provides sufficient statistics for any further study. Supported by several data types viz interaction data, expression data, Boolean data, and raw sequence data, modules and motifs provide an easy way to understand the specific function of a gene and protein. Basically, network motifs are characteristic network patterns comprising of both transcription regulation and protein-protein interaction that recur more often than in a random network.
The idea of network motif (sub-graph) was presented by Uri Alon and his group in 2002 [1] as they were discovered in a gene regulation network of E. coli and then in a large set of a neural network. According to their occurrence and behavior in a network, “motifs are subgraph recurring repeatedly, defined by a particular pattern of interaction between vertices that reflect a framework in which particular functions are achieved”. They are of vital importance largely because they may display functional properties and may also provide deep insight into network’s functional abilities. Significant studies have been done from perspective of the biological application as well as computational theory. Biological analysis mainly endeavors to interpret the functions of network motifs associated with genetic regulation as the first motif was found in the transcription unit of E. coli as well as Yeast and other higher organisms. Apart from those of genetic regulation, some distinct motifs were also discovered from the neural network and protein interaction network (fig-1).
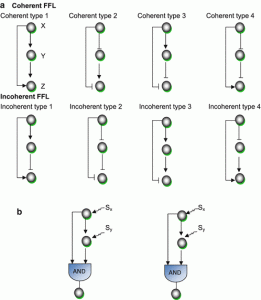
Statically a motif is identified as a pattern that occurs at least five times and is more significant than in a random network. With only two or at least three nodes, we may randomize to get a maximum pattern in a network. It is up to analyses that one has to perform. Patterns with two, three, four and five nodes are significant as their occurrence is more frequent in a network than any other pattern. Based on directivity, connectivity, pattern, regulation, and the number of nodes, they are classified into various categories as below:
1. Negative auto-regulation (NAR)
One of the simplest and most abundant network motifs is negative autoregulation in which a transcription factor represses its own transcription (fig. 2-a). Its generalized function is in response regulation and SOS DNA repair system response. NAR was observed to speed-up the response to signals in a synthetic transcription network. It also increases the stability of the auto-regulated gene product concentration against stochastic noises, reducing variations in protein levels between different cells.
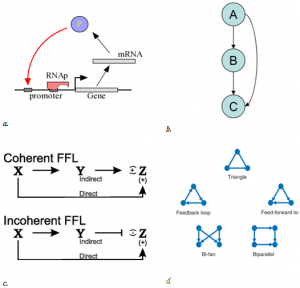
2. Positive auto-regulation (PAR)
It is characterized by the enhancement of transcription by its own gene product (fig 2-a). Comparatively, it shows a slower response than NAR. In a case where rapid regulation is required, PAR leads to a bimodal distribution of protein levels in cell populations.
3. Feed-forward loops (FFL)
This motif commonly occurs in many genetic regulatory networks and consists of three genes and three regulatory interactions (fig 2-b). In the diagram, target gene C is regulated by 2 TFs (transcription factor) A and B and in addition TF B is also regulated by TF A. Since each of the regulatory interaction may either be positive or negative, there are eight possible types of FFL motifs. Computationally, in most of the cases, FFL represent an AND & OR gate but other circuitry inputs are also possible.
4. Coherent type 1 FFL (C1-FFL)
This is one of the sub-types of FFL, characterized by giving a pulse filtration in which a short pulse of a signal will not generate a response but a persistent response will generate a short delay. Importantly, the signal response is fastened after one shut off. Such a vital mode of signal transduction in genetic or cellular regulatory system is observed in metabolic pathways and protein-gene interaction network.
5. Incoherent type 1 FFL (I1-FFL)
It is known to be a pulse generator and response accelerator. Two signal pathways function in two opposite ways, one signal activates and the other represses. After repression, a pulse dynamics is generated. Importantly, it speeds up the activation of any gene, not necessarily a gene of a transcription factor. Feedforward regulation shows better regulation than negative feedback.
6. Multi-output FFLs
The same regulator controls (regulates) multiple genes of the same system.
7. Single-input modules (SIM)
This motif occurs when a single gene regulates a single set of a gene with no additional regulation. This is significant when genes are carrying out a specific function and therefore need to be activated in the synchronized manner.
A possible confirmation of motif importance is motif conservation. In evolution conservation implies importance. The conservation of a protein in the network may be taken as an indication of the biological importance of that motif. The conservation of a motif shows the evolutionary pressure that can be followed to find ortholog in other organism. Wuchty et al, 2003 [2] tested this hypothesis for the correlation between the protein evolutionary rate and the structure of the motif it is embedded in. The conservation of motif component was found to be tens to thousands of times higher than expected at random, suggesting conservation of motif constituents. Motifs representing small functional unit or sub-graph in a network are found using different software like M-finder (http://www.weizmann.ac.il/mcb/Uri Alon/groupNetworkMotifSW.htm), MODIS, FANMOD (http://theinf1.informatik.uni-jena.dewernicke/motifs/index.htm), MAVisto (http://mavisto.ipk-gatersleben.de) iGRAPH: (https://cran.r-project.org/package=igraph), HOMER & Motif-X etc. Online tools like Amadeus, Web Motif, and MEME suite are also used for the same purpose.
References:
1. U Alon, Network Motif: theory and experimental approaches, Nature Reviews Genetics 8, 450-461,(June 2007) doi :10.1038
2. Wuchty S, et al. (2003) Evolutionary conservation of motif constituents in the yeast protein interaction network. Nat Genet 35(2):176-92.