Algorithms
miRBase: Explained
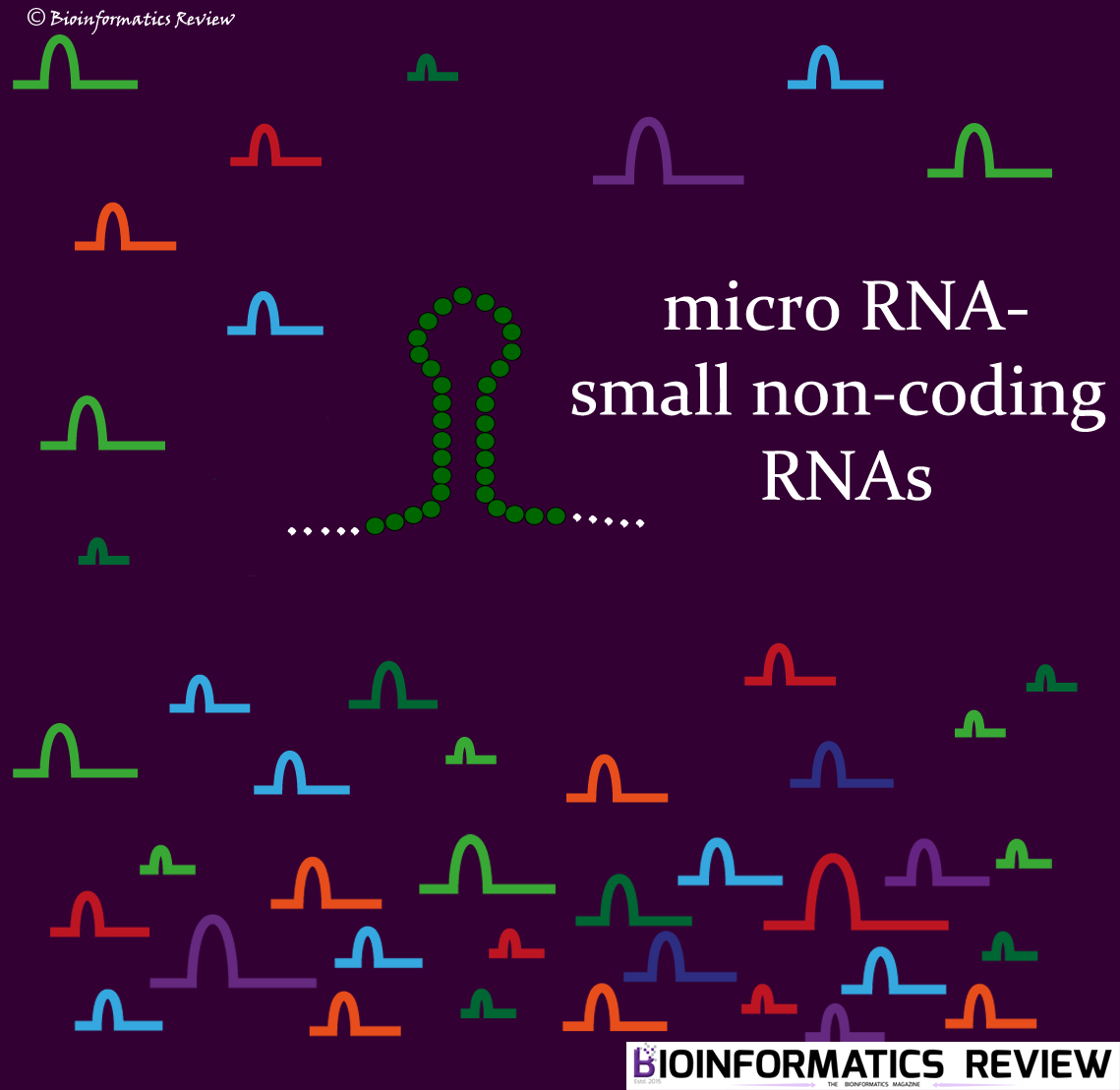
Micro RNAs (miRNAs) are the short endogenous RNAs (~22 nucleotides) and originate from the non-coding RNAs [1], produced in single-celled eukaryotes, viruses, plants, and animals [2]. miRNAs are capable of controlling homeostasis [2] and play significant roles in various biological processes such as degradation of mRNA and post-translational inhibition through complementary base pairing [3]. There are several miRNA databases which provide detailed information about the miRNA sequences, annotations, functions, and their predicted targets, among which miRBase is a primary online database for miRNA mature sequences and annotations [4-6]. This article explains the detailed structure and algorithm of miRBase.
miRBase is an online database which is available at www.mirbase.org [4-6]. The data can be downloaded from an FTP site (ftp://mirbase.org/pub/mirbase/CURRENT/) in different formats including FASTA and MySQL relational database dumps [7]. It provides a user-friendly interface to miRNA sequence data, its predicted gene targets, and annotations [6]. miRBase release 10.1 consists of 5071 miRNA loci from 58 species which expresses 5922 mature miRNA sequences [7]. miRBase has three main functions:
1. miRBase Registry:
It is a confidential source for assigning independent names to the novel miRNA genes even before their publication in any peer-reviewed journal [7]. This service is being used by over 70 publications. According to it, sequential numerical identifiers are assigned to the miRNAs, which uses 3 or 4 letters abbreviation to designate the species. For example, hsa-miR-101 (in Homo sapiens) [8].
2. miRBase Sequences:
It acts as a primary online repository for miRNA sequences and annotation. It provides the miRNA information, annotation, references, and links to other resources for all published and validated miRNAs [5,7]. This database consists of over 5000 sequences from 58 species.
3. miRBase Targets:
It is the database of predicted miRNA target genes. It predicts the targets for all published animal miRNAs [5,7]. The version 5 of this database predicts targets for over 5,00,000 mRNAs for all miRNAs in 24 different species. All individual miRNA-target binding sites, multiple conserved sites in the species, and multiple binding sites in UTRs are assigned a P-value [9], which helps the user to determine confidence in the predicted results.
miRBase has a nomenclature scheme for all predicted targets, its primary features are described as follows [7]:
- the predicted miRNA name composed of three or four alphabets species name as the prefix and a number as the suffix. For example, has-mir-212.
- A predicted mature miRNA sequence expressed from one or more hairpin precursor locus, composed of further numeric suffixes. For example, dme-mir-6-1 and dme-mir-6-2
- Related mature miRNA sequences expressed from the related hairpin loci, consists of further alphabets in their suffixes. For example, mmu-mir-181a and mmu-mir-181b.
- Plant miRNA genes are named as ath-MIR166a, where alphabets in the suffixes denote the distinct loci expressing all related mature miRNAs, and numbers are not used in the suffixes.
- Viral miRNA names consist of the locus from which they are derived. For example, ebv-mirBART1 from the Epstein-Barr virus BART locus.
miRBase Data
The latest release of miRBase (release 20) has updated the database with 24,521 hairpin sequences from 206 species, and 30424 mature sequences [10]. In many cases, the 5’ and 3’ arm of the hairpin precursor expresses the mature miRNAs suggesting that either both may be functional, or there is no sufficient data available to determine the predominant product [7]. Such miRNAs are depicted as has-miR-140-5p and has-miR-140-3p. The ‘Evidence’ field provides information about the origin of each sequence available in the database.
The miRBase:Targets database predict targets in the UTRs of 37 different animal genomes from Ensembl [5,7]. miRBase provides a list of mRNAs overlapping each miRNA defining its type (intron, UTR or exon) and the sense (forward or reverse) [7]. miRNAs are often clustered within a genome, therefore, miRBase provides a list of such miRNAs which can be retrieved for any organism. miRBase also displays the distribution of genomic features of miRNAs, showing CpG islands, poly-A site, EST, cDNA, TSS, and DITAGs. TSSs are predicted using the Eponine-TSS software.
How does it work?
miRBase uses the miRANDA algorithm [7,11] to identify all available miRNA sequences for a particular genome against 3’-UTR of that genome obtained from Ensembl [12]. The algorithm is based on dynamic programming which searches for maximum local complementarity alignments. For every pair of G:C and A:T, a score of +5 is assigned, for G:U wobble pairs, +2 is assigned, and -3 for mismatch pair, also the gap opening and gap elongation parameters are set to −8.0 and −2.0, respectively [11,12]. It calculates the optimal alignment score at the positions i, j by forming an alignment scoring matrix. The gap-elongation parameter has been used only if the extension to i, j of a given stretch of gaps ending at positions i–1, j or j–1, i (but not of stretches of gaps ending at i–k, j or j, i–k for k > 1) resulted in a higher score than the addition of a nucleotide-nucleotide match at positions i, j. Complementarity scores at the first eleven residues of the miRNA 5’-end, are multiplied by a scaling factor of 2.0 to achieve the experimentally observed 5’-3’ asymmetry, e.g., G:C and A:T base pairs contributed +10 to the match score in these positions. This value of scaling factor is adjustable. There are few rules for the target prediction: the threshold for candidate target site is S > 90 and ΔG < −17 kcal/mol, where S is the sum of single pair of matching residue scores over the alignment trace and ΔG is the free energy of duplex formation from a completely dissociated state [11,12].
The algorithm finds the optimal local matches above this threshold between a particular miRNA and a set of 3’ UTRs in each genome, after that it checks whether the sequence of this miRNA and target site position is conserved in orthologous genes, i.e., human, mouse, or rat, or fugu, and zebrafish [12]. The alignment between the target sites is transitive in nature (UTR to miRNA to UTR) through a homologous miRNA. It is necessary that the positions of the target sites pairs should fall between ±10 residues of the aligned UTRs. When this criterion gets fulfilled, the conserved target sites with 90% or more sequence identity (human versus mouse or rat) and 70% or more (fugu versus zebrafish), are selected as the candidate miRNA target sites and stored in the database (MYSQL) [11]. John et al., 2005, has predicted 10,572 target sites which are conserved in either mouse or rat in 4,463 human transcripts, of which 2,307 transcripts of 2,273 genes contained more than one target site. Similarly, using zebrafish as a reference species, they predicted 7,057 conserved target sites (conserved in fugu) in 4,820 zebrafish transcripts [12].
The conserved target sites for each miRNA are sorted according to the alignment score, in which free energy acts as the secondary sort criterion. When a single site of a mRNA (or within 25 nts) is targeted by multiple miRNAs, the miRNA having the highest scoring lowest energy is reported for that site [11].
Recently, MiRBase has introduced high-confidence miRNAs based on the pattern of deep-sequencing data [10]. In order to be classified under high-confidence miRNAs, a locus must fulfill the following criteria [10]:
- a minimum of 10 reads must map to the two possible mature miRNAs obtained from the hairpin precursor.
- a minimum of half of the reads mapping to each arm of the hairpin precursor must consist of the same 5′-end.
- a minimum or more than half fo the bases (60%) in mature sequences must pair with the predicted hairpin structure.
- the predicted hairpin structure must have a folding free energy of <0.2 kcal/mol/nt.
- the most abundant reads present at each arm of the hairpin precursor must be paired to the mature miRNA duplex with 0-4 nucleotides at their 3′-ends.
This was all about miRBase if you would like to read more about miRNA prediction using deep sequencing data, click here. We will be discussing other miRNA databases in detail in the upcoming articles.
References
- Bartel, D. P. (2004). MicroRNAs: genomics, biogenesis, mechanism, and function. cell, 116(2), 281-297.
- Liu, B., Li, J., & Cairns, M. J. (2012). Identifying miRNAs, targets and functions. Briefings in bioinformatics, 15(1), 1-19.
- He, L., & Hannon, G. J. (2004). MicroRNAs: small RNAs with a big role in gene regulation. Nature Reviews Genetics, 5(7), 522.
- Griffiths-Jones, S. (2006). miRBase: the microRNA sequence database. In MicroRNA Protocols (pp. 129-138). Humana Press.
- Griffiths-Jones, S., Grocock, R. J., Van Dongen, S., Bateman, A., & Enright, A. J. (2006). miRBase: microRNA sequences, targets and gene nomenclature. Nucleic acids research, 34(suppl_1), D140-D144.
- Griffiths‐Jones, S. (2010). miRBase: microRNA sequences and annotation. Current protocols in bioinformatics, 29(1), 12-9.
- Griffiths-Jones, S., Saini, H. K., van Dongen, S., & Enright, A. J. (2007). miRBase: tools for microRNA genomics. Nucleic acids research, 36(suppl_1), D154-D158.
- Griffiths‐Jones, S. (2004). The microRNA registry. Nucleic acids research, 32(suppl_1), D109-D111.
- Rehmsmeier, M., Steffen, P., Höchsmann, M., & Giegerich, R. (2004). Fast and effective prediction of microRNA/target duplexes. Rna, 10(10), 1507-1517.
- Kozomara, A., & Griffiths-Jones, S. (2013). miRBase: annotating high confidence microRNAs using deep sequencing data. Nucleic acids research, 42(D1), D68-D73.
- Enright, A. J., John, B., Gaul, U., Tuschl, T., Sander, C., & Marks, D. S. (2003). MicroRNA targets in Drosophila. Genome biology, 5(1), R1.
- John B, Enright AJ, Aravin A, Tuschl T, Sander C, et al. (2005) Correction: Human MicroRNA Targets. doi: info:doi/10.1371/journal.pbio.0030264
Algorithms
MOCCA- A New Suite to Model cis- regulatory Elements for Motif Occurrence Combinatorics
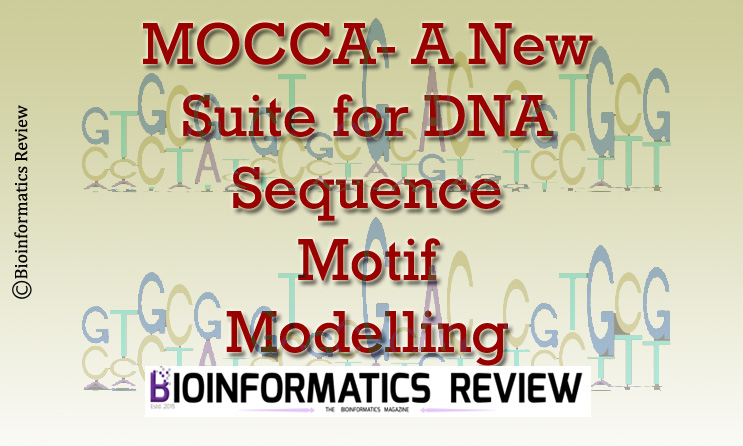
cis-regulatory elements are DNA sequence segments that regulate gene expression. cis-regulatory elements consist of some regions such as promoters, enhancers, and so on. These regions consist of specific sequence motifs. (more…)
Algorithms
vs_Analysis.py: A Python Script to Analyze Virtual Screening Results of Autodock Vina
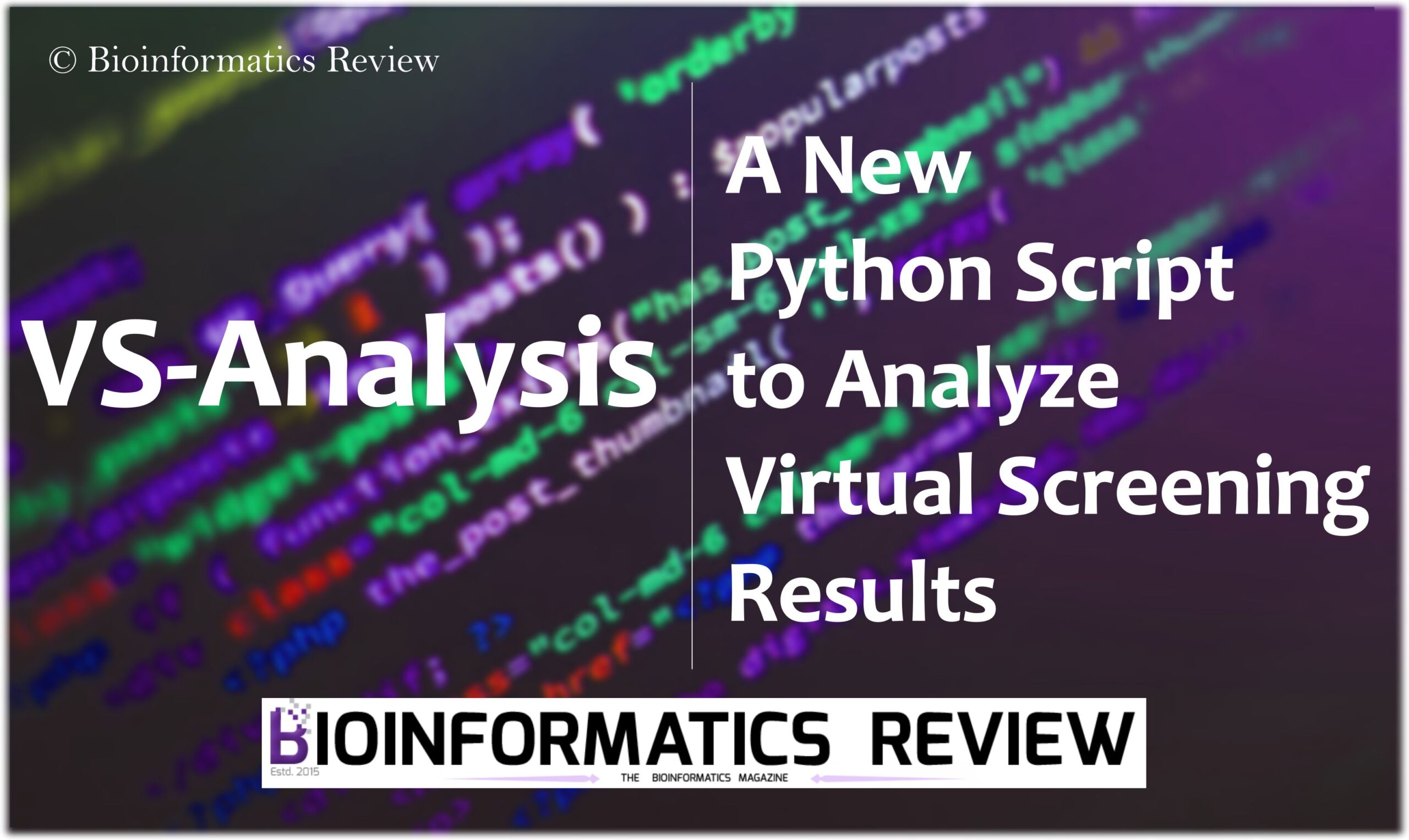
The output files obtained as a result of virtual screening (VS) using Autodock Vina may be large in number. It is difficult or quite impossible to analyze them manually. Therefore, we are providing a Python script to fetch top results (i.e., compounds showing low binding affinities). (more…)
Algorithms
How to search motif pattern in FASTA sequences using Perl hash?
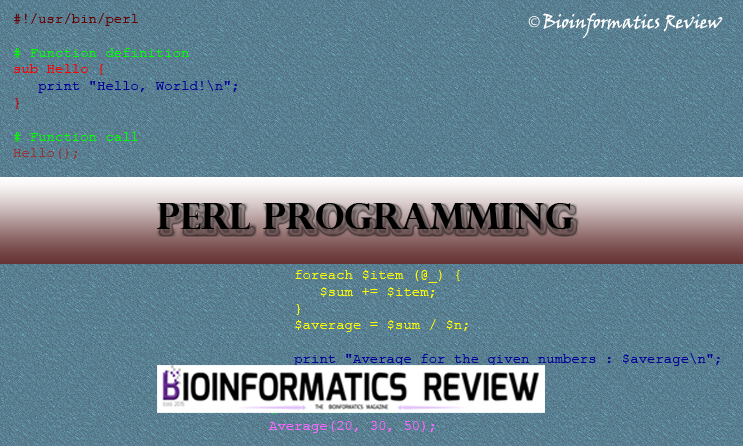
Here is a simple Perl script to search for motif patterns in a large FASTA file with multiple sequences.
Algorithms
How to read fasta sequences from a file using PHP?
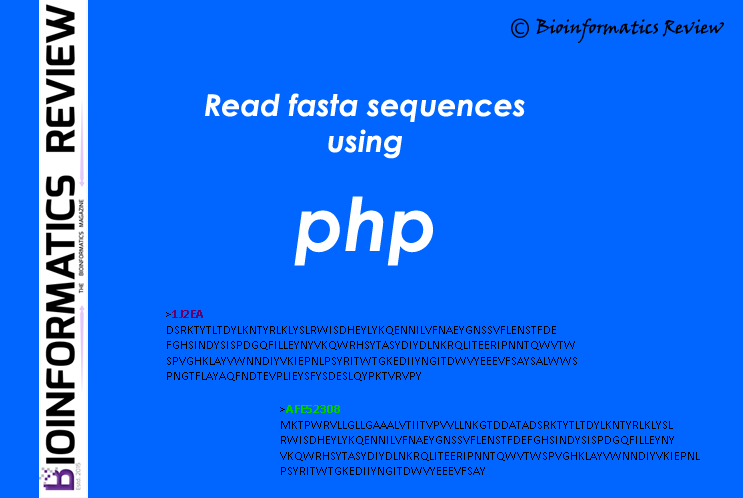
Here is a simple function in PHP to read fasta sequences from a file. (more…)
Algorithms
How to read fasta sequences as hash using perl?
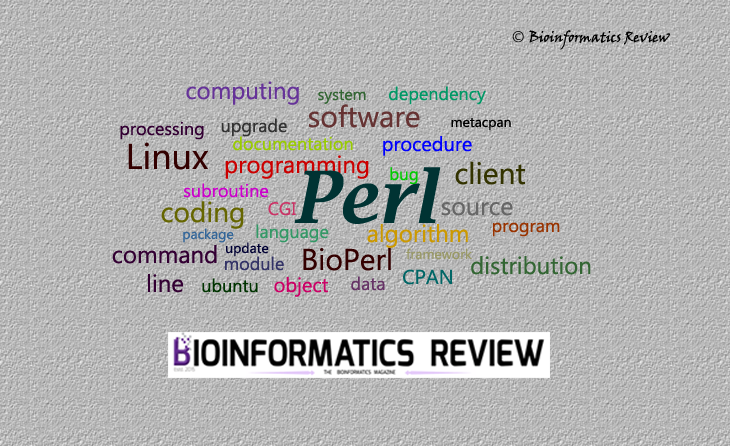
This is a simple Perl script to read a multifasta file as a hash. (more…)
Algorithms
BETSY: A new backward-chaining expert system for automated development of pipelines in Bioinformatics
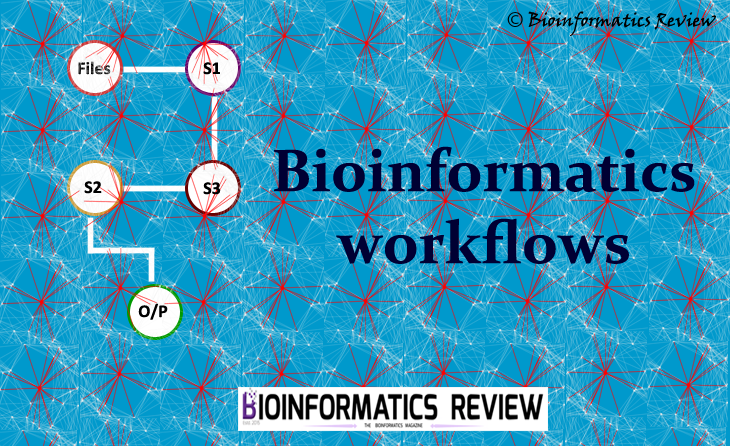
Bioinformatics analyses have become long and difficult as it involves a large number of steps implemented for data processing. Bioinformatics pipelines are developed to make this process easier, which on one hand automate a specific analysis, while on the other hand, are still limited for investigative analyses requiring changes to the parameters used in the process. (more…)
Algorithms
Algorithm and workflow of miRDB
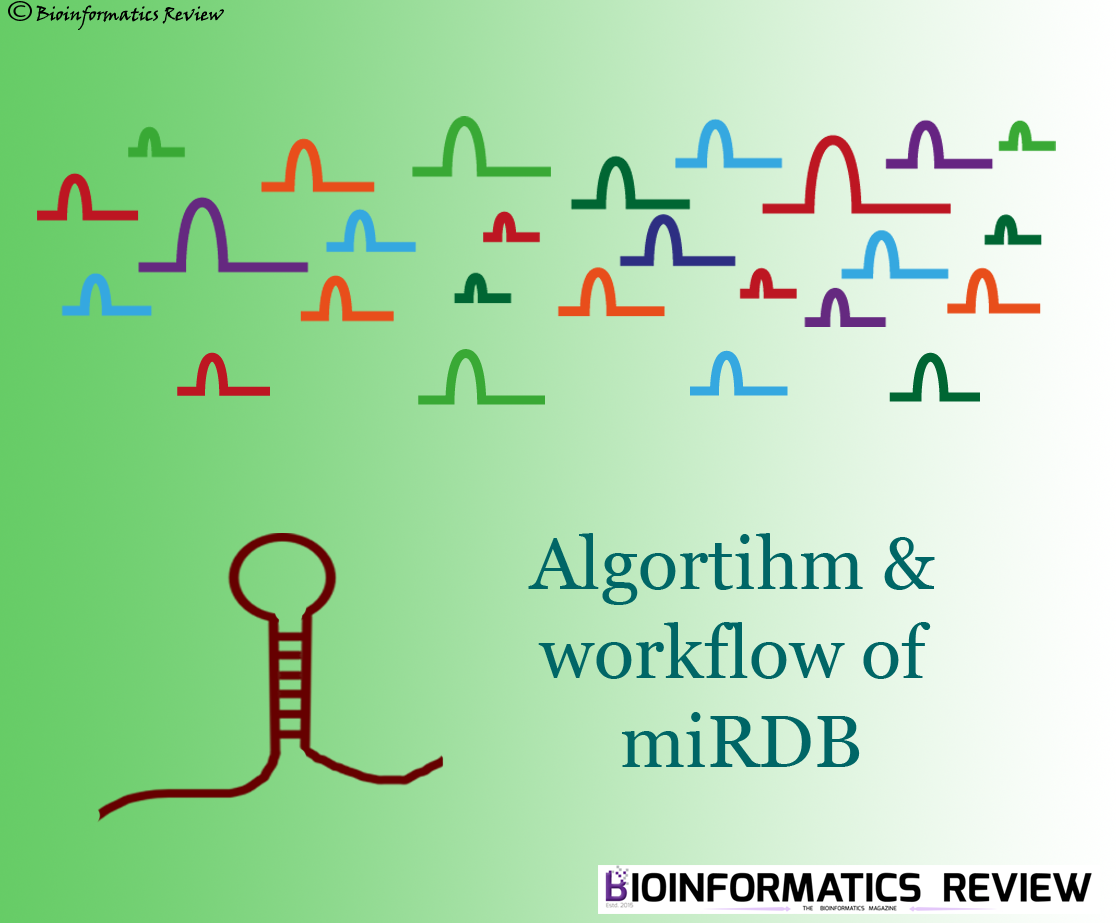
As mentioned in the previous article, Micro RNAs (miRNAs) are the short endogenous RNAs (~22 nucleotides) and originate from the non-coding RNAs [1], produced in single-celled eukaryotes, viruses, plants, and animals [2]. They play significant roles in various biological processes such as degradation of mRNA [3]. Several databases exist storing a large amount of information about miRNAs, one of such databases miRBase [4] was explained in the previous article, today we will explain the algorithm of miRDB [5,6], another database for miRNA target prediction. (more…)
Algorithms
Prediction of biochemical reactions catalyzed by enzymes in humans
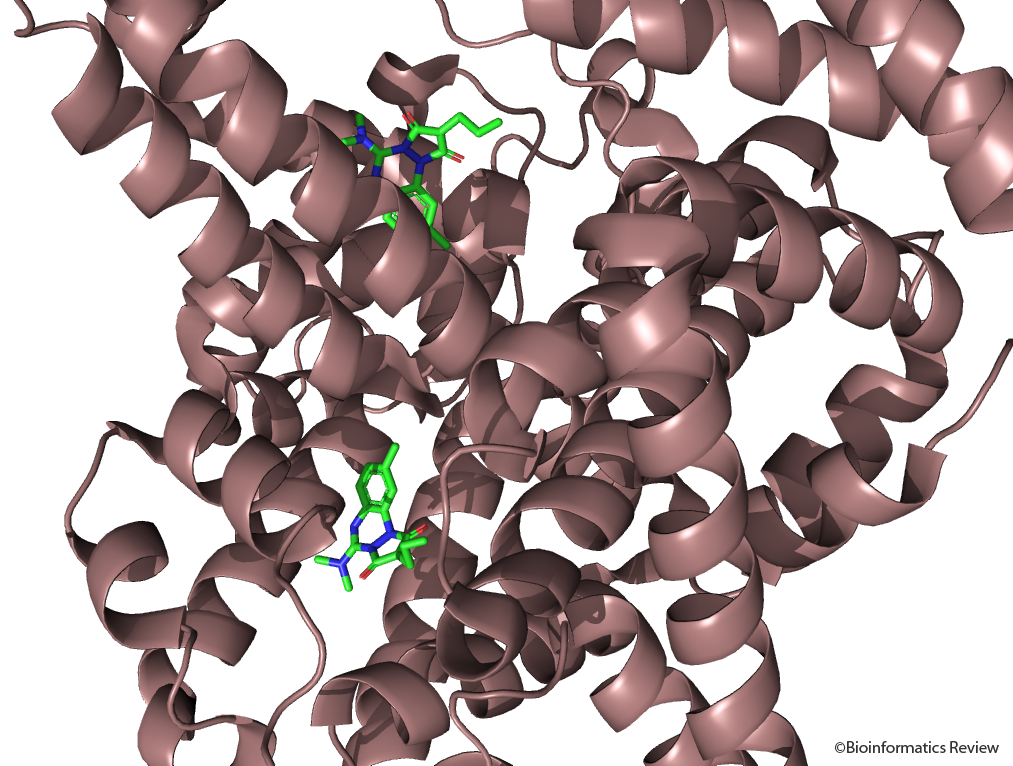
There are many biological important enzymes which exist in the human body, one of them is Cytochrome P450 (CyP450) enzymes which are mostly considered in drug discovery due to their involvement in the majority (75%) of drug metabolism [1]. Therefore, various in-silico methods have been applied to predict the possible substrates of CyP 450 enzymes [2-4]. Recently, an in-silico model has been developed to predict the potential chemical reactions mediated by the enzymes present in humans including CyP450 enzymes [5]. (more…)
Algorithms
A new high-level Python interface for MD simulation using GROMACS
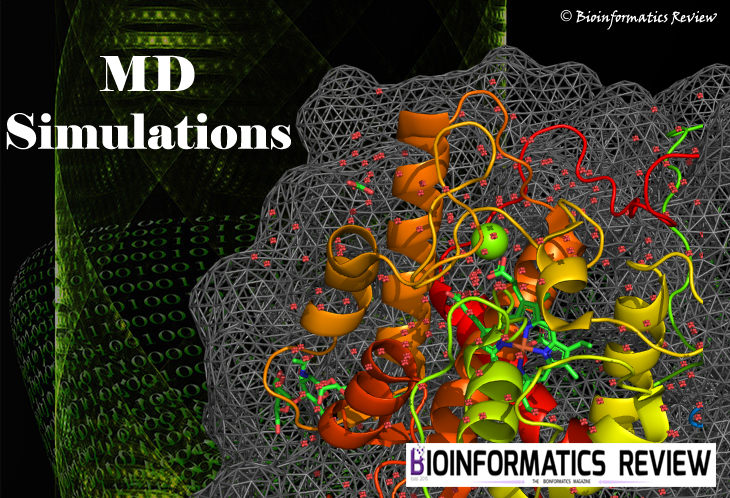
The roots of the molecular simulation application can be traced back to physics where it was applied to simplified hard-sphere systems [1]. This field of molecular simulation study has gained a lot of interest since then and applied to perform simulations to fold small protein at multi-microsecond scale [2-4], predict functional properties of receptors and to capture the intermediate transitions of the complex [5], and to study the movement and behavior of ligand in a binding pocket and also to predict interactions between receptors and ligands [6,7]. (more…)
Algorithms
Machine learning in prediction of ageing-related genes/proteins
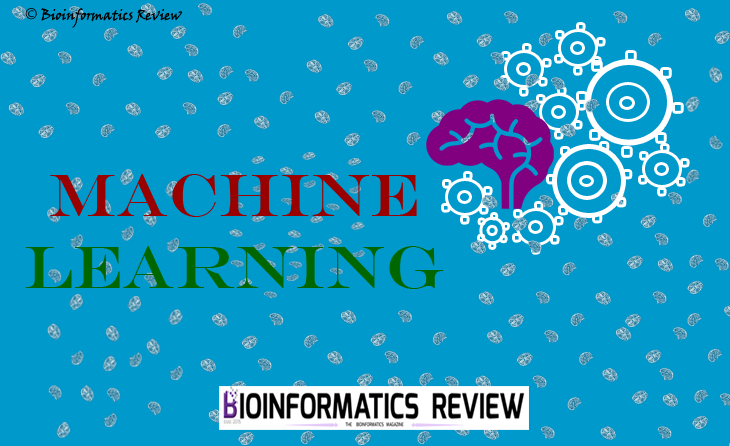
Ageing has a great impact on human health, when people’s age advance towards 80 years, approximately half of the proteins in the body get damaged through oxidation. The chemical degradations occurring in our body produce energy by the consumed food via oxidation in the presence of oxygen. (more…)
Algorithms
Simulated sequence alignment software: An alternative to MSA benchmarks
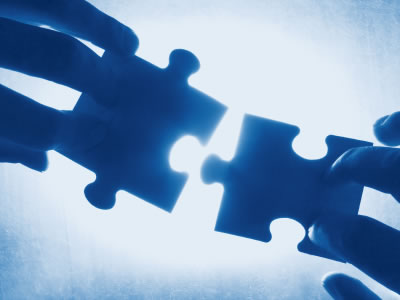
In our previous article, we discussed different multiple sequence alignment (MSA) benchmarks to compare and assess the available MSA programs. However, since the last decade, several sequence simulation software have been introduced and are gaining more interest. In this article, we will be discussing various sequence simulating software being used as alternatives to MSA benchmarks. (more…)
Algorithms
Benchmark databases for multiple sequence alignment: An overview
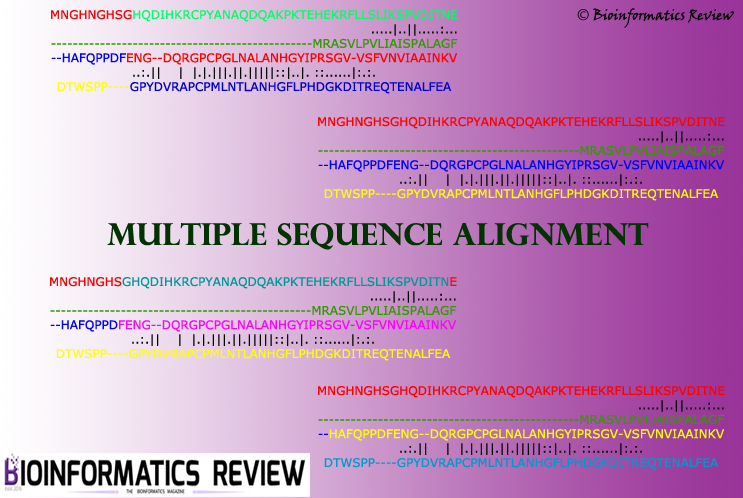
Multiple sequence alignment (MSA) is a very crucial step in most of the molecular analyses and evolutionary studies. Many MSA programs have been developed so far based on different approaches which attempt to provide optimal alignment with high accuracy. Basic algorithms employed to develop MSA programs include progressive algorithm [1], iterative-based [2], and consistency-based algorithm [3]. Some of the programs incorporate several other methods into the process of creating an optimal alignment such as M-COFFEE [4] and PCMA [5]. (more…)
Algorithms
ab-initio prediction of protein structure: An introduction
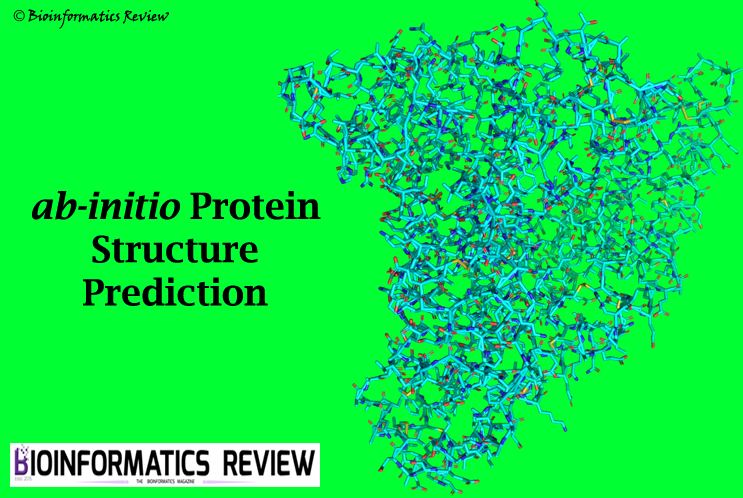
We have heard a lot about the ab-initio term in Bioinformatics, which could be difficult to understand for newbies in the field of bioinformatics. Today, we will discuss in detail what ab-initio is and what are the applicable methods for it. (more…)
Algorithms
Intrinsically disordered proteins’ predictors and databases: An overview
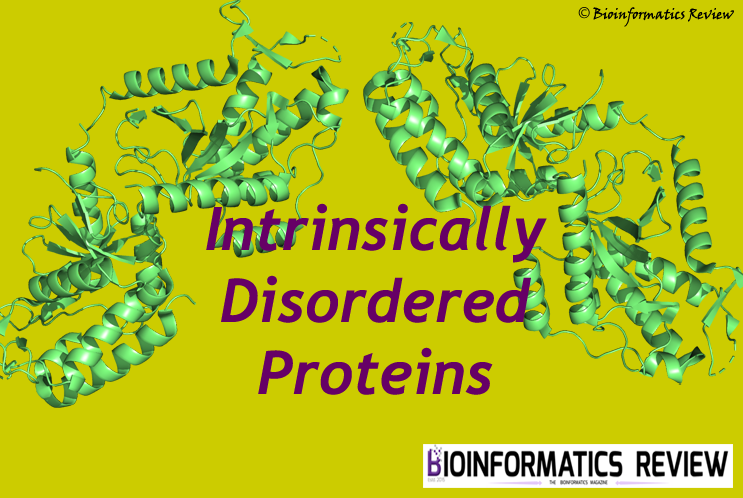
Intrinsically unstructured proteins (IUPs) are the natively unfolded proteins which must be unfolded or disordered in order to perform their functions. They are commonly referred to as intrinsically disordered proteins (IDPs) and play significant roles in regulating and signaling biological networks [1]. IDPs are also involved in the assembly of signaling complexes and in the dynamic self-assembly of membrane-less nuclear and cytoplasmic organelles [1]. The disordered regions in a protein can be highly conserved among the species in respect of both the composition and the sequence [2]. (more…)
Algorithms
An introduction to the predictors of pathogenic point mutations
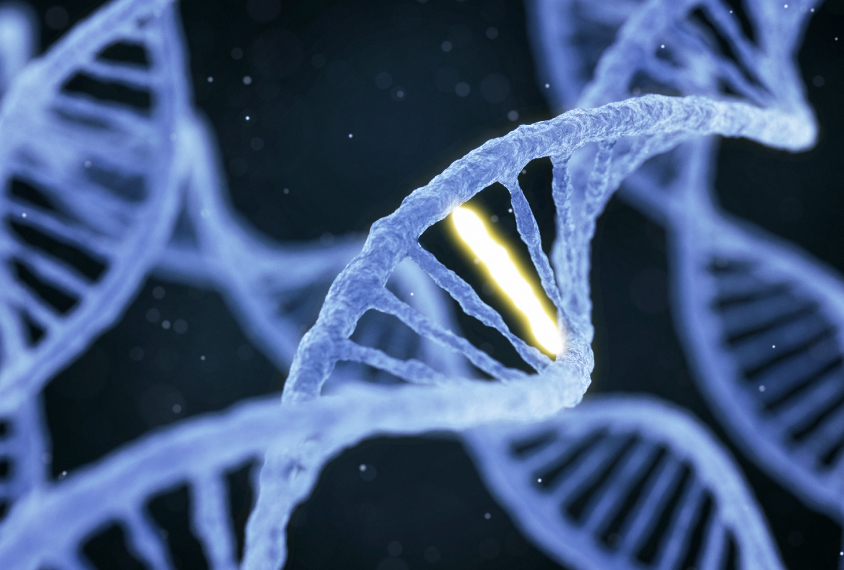
Single nucleotide variation is a change in a single nucleotide in a sequence irrespective of the frequency of the variation. Single nucleotide variants (SNVs) play a very important role in causing several diseases such as the tumor, cancer, etc. Many efforts have been made to identify the SNVs which were initially based on identifying non-synonymous mutations in coding regions of the genomes. (more…)
Algorithms
SparkBLAST: Introduction
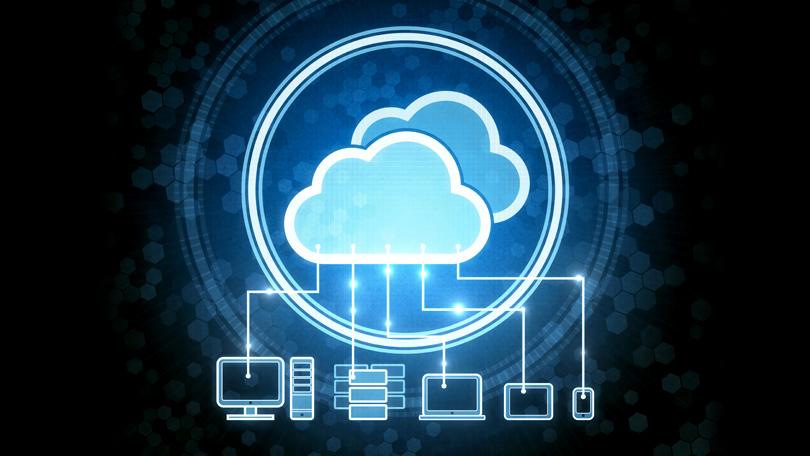
The basic local alignment search tool (BLAST) [1,2] is known for its speed and results, which is also a primary step in sequence analysis. The ever-increasing demand for processing huge amount of genomic data has led to the development of new scalable and highly efficient computational tools/algorithms. For example, MapReduce is the most widely accepted framework which supports design patterns representing general reusable solutions to some problems including biological assembly [3] and is highly efficient to handle large datasets running over hundreds to thousands of processing nodes [4]. But the implementation frameworks of MapReduce (such as Hadoop) limits its capability to process smaller data. (more…)
Algorithms
Role of Information Theory, Chaos Theory, and Linear Algebra and Statistics in the development of alignment-free sequence analysis
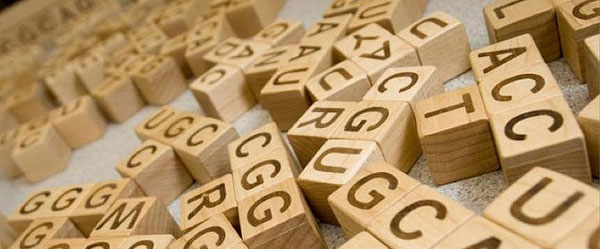
Sequence alignment is customary to not only find similar regions among a pair of sequences but also to study the structural, functional and evolutionary relationship between organisms. Many tools have been discovered to achieve the goal of alignment of a pair of sequences, separately for nucleotide sequence and amino acid sequence, BLOSSUM & PAM [1] are a few to name. (more…)
Algorithms
Bioinformatics Challenges and Advances in RNA interference
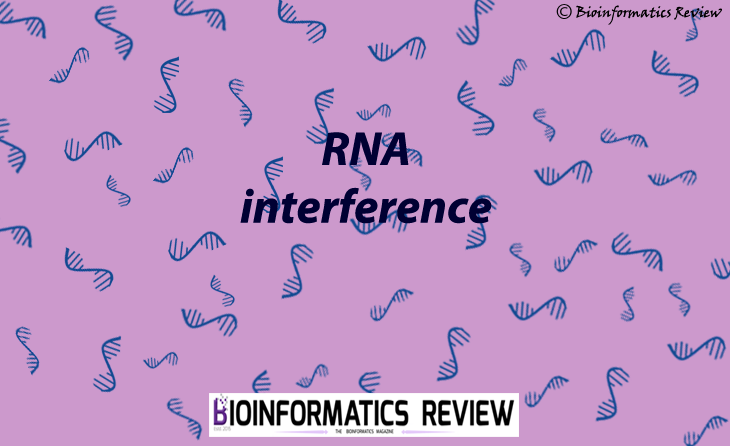
RNA interference is a post-transcriptional gene regulatory mechanism to down-regulate the gene expression either by mRNA degradation or by mRNA translation inhibition. The mechanism involves a small partially complementary RNA against the target gene. To perform the action, it also requires a class of dedicated proteins to process these primary RNAs into mature microRNAs. The guide sequence determines the specificity of the miRNA. Therefore, the knowledge of the guide sequence is crucial for predicting its targets and also exploiting the sequence to create a new regulatory circuit. In this short review, we will briefly discuss the role and challenges in miRNA research for unveiling the target prediction by bioinformatics and to foster our understanding and applications of RNA interference. (more…)
Algorithms
Systems pharmacology and drug development
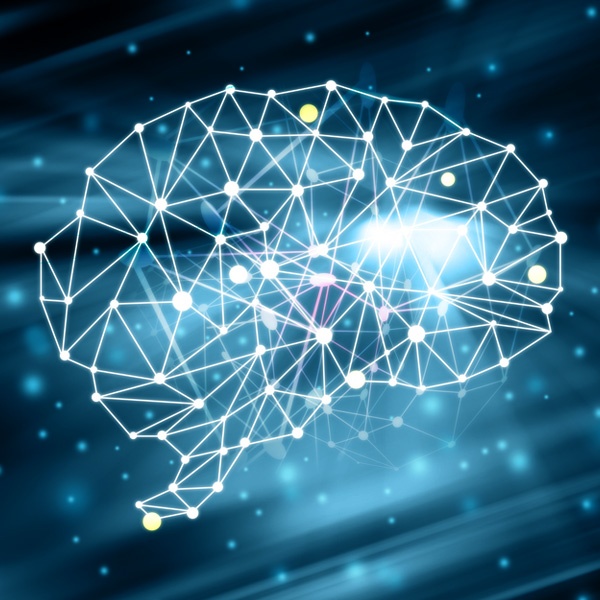
Systems pharmacology is an emerging area in the field of medicinal chemistry and pharmacology which utilizes systems network to understand drug action at the organ and organism level. It applies the computational and experimental systems biology approaches to pharmacology, which includes network analyzes at multiple biological organization levels facilitating the understanding of both therapeutic and adverse effects of the drugs. Nearly a decade ago, the term systems pharmacology was used to define the drug action in a specific organ system such as reproductive pharmacology [1], but to date, it has been expanded to different organ and organism levels [2]. (more…)
Algorithms
Recent advances in in-silico approaches for enzyme engineering
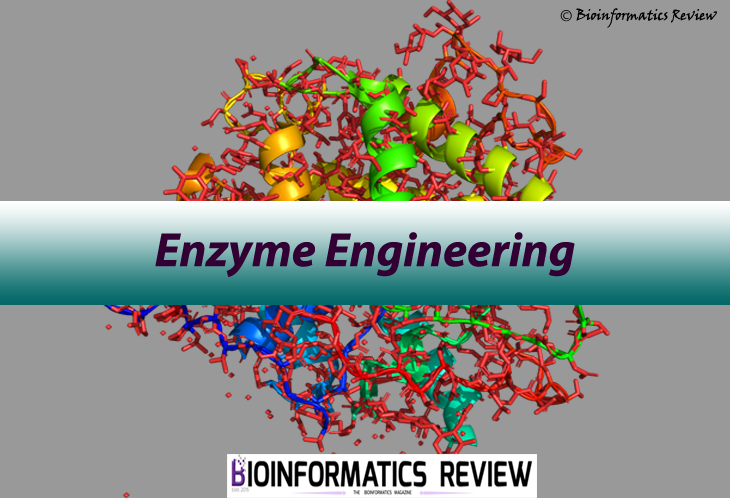
Enzymes are natural biocatalysts and an attractive alternative to chemicals providing improved efficiency for biochemical reactions. They are widely utilized in industrial biotechnology and biocatalysis to introduce new functionalities and enhance the production of enzymes. In order to be proved beneficial for industrial purposes, the enzymes need to be optimized by applying protein engineering. This article specifically reviews the recent advancements in the computational approaches for enzyme engineering and structural determination of the enzymes developed in recent years to improve the efficiency of enzymes, and the creation of novel functionalities to obtain
products with high added value for industrial applications. (more…)
You must be logged in to post a comment Login