HTS
Assembly of high-throughput mRNA-Seq data: A review
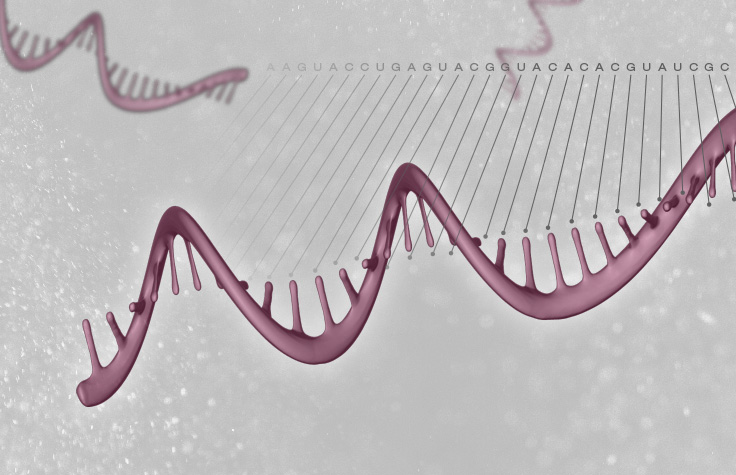
Transcriptome represents the complete set of all expressed transcripts (RNA molecules) present in a cell or tissue at a given point of time. The transcriptome is always dynamic in nature and keeps on changing with time driven by the external and internal environment. We know that among the total transcribed RNA transcripts, only a small fraction is translated into proteins. The fraction which is translated into proteins is referred to as coding transcriptome, while the fraction which is not translated is referred to as non-coding transcriptome. In other words, coding transcriptome is a collection of all the messenger RNA (mRNA) molecules while non-coding transcriptome is a repertoire of transfer RNA (tRNA), ribosomal RNA (rRNA), small nuclear and small nucleolar RNA (sn/snoRNA) and small RNAs (siRNA, miRNA, lsiRNA).
In past, a number of technologies have been developed to investigate the expression of genes. One way of doing this is to capture the expressed mRNA transcripts and evaluate relative abundance against different conditions. mRNA-Seq is a recently developed high-throughput method which works on the genome-wide level. In this method, total RNA is first extracted from control and test tissue/s or cell which is subsequently used to fish mRNA population using an oligo-dT probe and A-tail attached to mRNA transcripts. This mRNA population is fragmented, size selected (according to the need of the sequencing approach – single read or paired end) and reverse transcribed to obtain a cDNA library. Later on, sequencing adapters were ligated and the adapter-ligated cDNA library was again size selected. Before sequencing, a few cycles of PCR were used to enrich the cDNA library concentration. The mRNA-Seq libraries can be sequenced through single-read or paired-end read approach. In single read format, a DNA fragment is sequenced for a particular length from one end only. On the other hand, in a paired-end format, a DNA fragment is sequenced from both ends for a defined length. Paired-end sequencing is a preferred method of sequencing as it yields more sequencing data which results in greater coverage. After cleaning of data (removal of low-quality reads), the reads (paired or unpaired) can be assembled computationally.
Next-generation sequencing of mRNA-Seq libraries results in millions of reads. It is a computationally challenging task to stitch these reads into an actual transcriptome. Presently, various assemblers such as Velvet-oases, Trinity, SOAPdenovo-Trans, Trans-ABySS, ALLPATHS-LG etc. are available to suit different needs of assembly [1, 12, 10, 2, 5]. However, it is still very difficult to create a high confidence error-free assembly. In the following section, popular strategies used for computational reconstruction of sequencing reads into a functional transcriptome are discussed:
Reference-based assembly:
In this method, purified sequencing reads are first mapped to a genome of the same or related species. That is, the computational assembly is built upon a reference genomic platform and hence referred to as reference-based assembly [3]. Succeeding the mapping step, the sequencing reads which map to identical locus are independently clustered and thereafter traversed to identify different genes and their isoforms. There are several advantages of using reference-based assembly. For example, even scarcely abundant expression (of only a few reads) can be detected, overall assembly has less number of contaminants/artifacts, errors and a high confidence. Gaps in the assembled draft can be easily filled with the information of reference genome or transcriptome. Moreover, it is also possible to predict transcription start and stop sites. Transcriptomes of polyploidy crop plants are more prone to error due to their high sequence similarity among different genes and thus often result in miss-assembly of entirely different transcripts as one transcript [9, 11, 7].
de-novo assembly:
For most of the organisms genome information is still lacking. In such cases, where a reference is not available, a de-novo method is utilized to develop an assembly. Here, multiple overlapping sequencing reads are clustered as contigs which are further reconstructed as entire transcriptome. This approach is practiced most of the time as genome drafts are available for only a few organisms. However, for performing a de-novo assembly, a very high coverage of transcriptome is required which in turn requires performing several sequencing runs to generate required sequencing depth. On the other hand, in reference-based assembly, even a low coverage (10X) can be used to produce high confidence assembly. It is also advisable to use different k-mer to identify optimal k-mer length which will be used to generate an assembly. Many researchers use multiple k-mers to develop multiple assemblies which are then merged into a single assembly [6]. On a different note, assemblies can be generated using different assemblers. These different assemblies are then searched for common transcripts which thereafter stitched as a single assembly [7,8].
References:
- Butler J, MacCallum I, Kleber M, Shlyakhter IA, Belmonte MK, Lander ES, Nusbaum C, Jaffe DB. 2008. ALLPATHS: de novo assembly of whole-genome shotgun microreads. Genome research 18: 810-820.
- Grabherr MG, Haas BJ, Yassour M, Levin JZ, Thompson DA, Amit I, Adiconis X, Fan L, Raychowdhury R, Zeng Q. 2011. Full-length transcriptome assembly from RNA-Seq data without a reference genome. Nature biotechnology 29: 644-652.
- Guttman M, Garber M, Levin JZ, Donaghey J, Robinson J, Adiconis X, Fan L, Koziol MJ, Gnirke A, Nusbaum C. 2010. Ab initio reconstruction of cell type-specific transcriptomes in mouse reveals the conserved multi-exonic structure of
- Nature biotechnology 28: 503-510
- Luo R, Liu B, Xie Y, Li Z, Huang W, Yuan J, He G, Chen Y, Pan Q, Liu Y. 2012. SOAPdenovo2: an empirically improved memory-efficient short-read de novo assembler. Gigascience 1: 18.
- Martin J, Bruno VM, Fang Z, Meng X, Blow M, Zhang T, Sherlock G, Snyder M, Wang Z. 2010. Rnnotator: an automated de novo transcriptome assembly pipeline from stranded RNA-Seq reads. BMC genomics 11: 663.
- Martin JA, Wang Z. 2011. Next-generation transcriptome assembly. Nature Reviews Genetics 12: 671-682.
- Nakasugi K, Crowhurst R, Bally J, Waterhouse P. 2014. Combining Transcriptome Assemblies from Multiple De Novo Assemblers in the Allo-Tetraploid Plant Nicotiana benthamiana. PloS one 9: e91776.
- Robertson G, Schein J, Chiu R, Corbett R, Field M, Jackman SD, Mungall K, Lee S, Okada HM, Qian JQ. 2010. De novo assembly and analysis of RNA-seq data. Nature methods 7: 909-912.
- Simpson JT, Wong K, Jackman SD, Schein JE, Jones SJ, Birol I. 2009. ABySS: a parallel assembler for short read sequence data. Genome research19: 1117-1123.
- Surget-Groba Y, Montoya-Burgos JI. 2010. Optimization of de novo transcriptome assembly from next-generation sequencing data. Genome research 20: 1432-1440.
- Zerbino DR, Birney E. 2008. Velvet: algorithms for de novo short read assembly using de Bruijn graphs. Genome research 18: 821-829.
You must be logged in to post a comment Login