Bioinformatics Programming
TIN: R package to analyze Transcriptome Instability
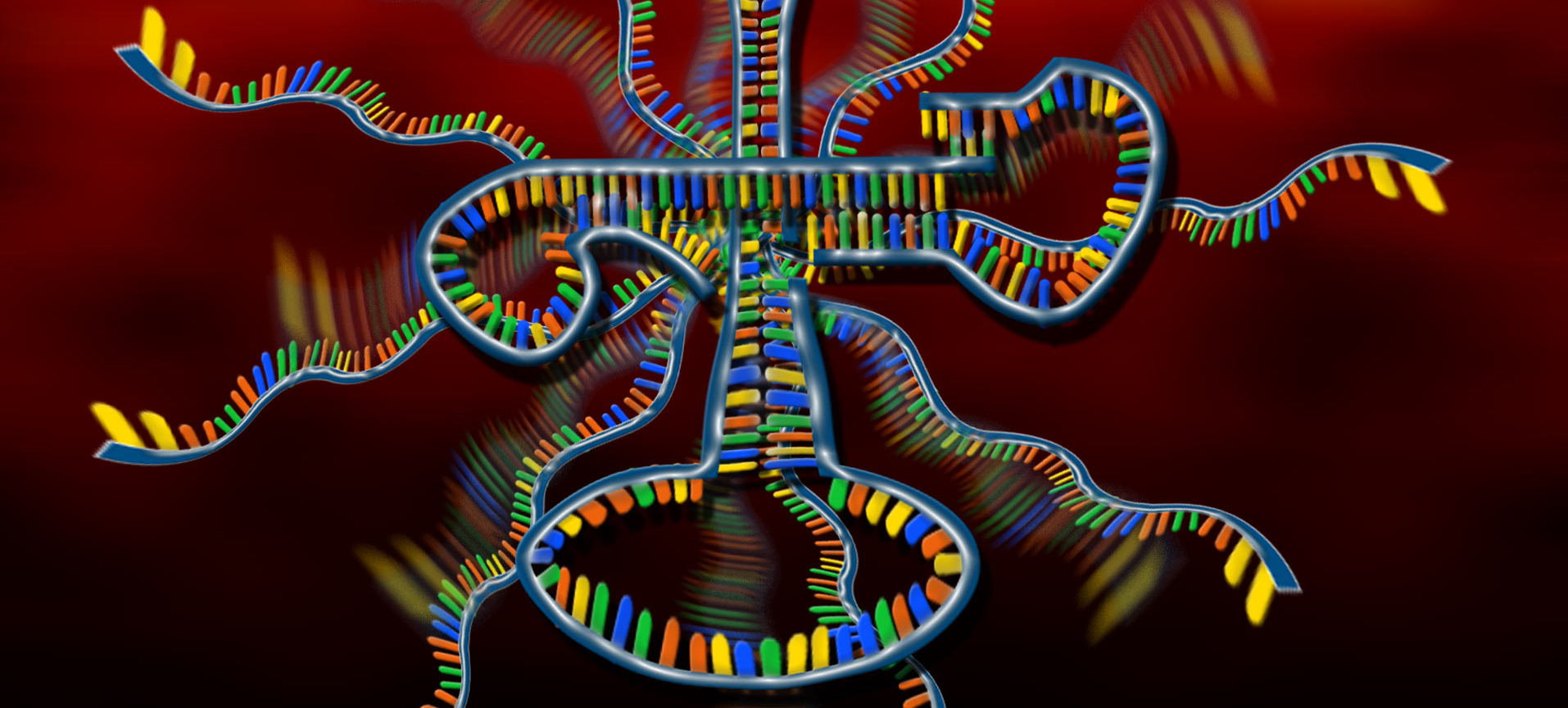
Alternative Splicing plays a very essential role in proper functioning of eukaryotic cells. It acts as a regulatory mechanism for gene expression and any kind of disruption in this mechanism may lead to human diseases. Alternative splicing of pre-mRNA is a major source of genetic variation in human beings and disruption of the splicing process may cause human diseases such as cancer. Cancer-associated variation which may occur at different levels of gene regulation, particularly during the processing of pre-mRNA into mature mRNAs. So, better understanding of these mechanisms may provide insights into disease causes and development.
TIN is a new R package which enables to analyze TIN from the expression data. TIN is a software package of R modules that uses a framework to analyze expression level data.
WORKFLOW:
TIN uses raw expression data (cell intensity,CEL files) as input and applies the FIRMA method (i.e., a method for detection of alternative splicing) estimating the expression levels of transcriptome and the alternative splicing patterns between samples. FIRMA method gives a FIRMA score to each exon sample combination, which is based on the deviation of probes systems from the expected gene expression level. Thus, FIRMA score is the relative ratio between exon expression level and corresponding gene expression level. If FIRMA shows a strong positive score, then the differential exon is included and if it shows a negative score, then it implies that exon is skipped.
Since alternative splicing is mediated by several splicing factors and proteins which remove introns from the pre-mRNA then joining the exons of mRNA together. Therefore, TIN basically test the association between splicing factor expression levels and amount of abnormal exon usage among the samples. For this, correlation between abnormal exon usage amounts and splicing factor expression levels tested across all samples is calculated. If the correlation is considerably lower, it indicates that the aberrant amounts of exon expression may be due to splicing factor expression. After that, correlation is tested by using random gene sets, if the correlation is poor then it gives an indication that the abnormal exon usage can be attributed to the expression levels of the splicing factor genes.
Fig.1 Workflow of TIN
This is how by analyzing the gene expression levels and alternative splicing patterns we can easily monitor a developing disease or it can be predicted at an very early stage.
For further reading, click here.
Note:
An exhaustive list of references for this article is available with the author and is available on personal request, for more details write to [email protected]
Bioinformatics Programming
How to create a pie chart using Python?
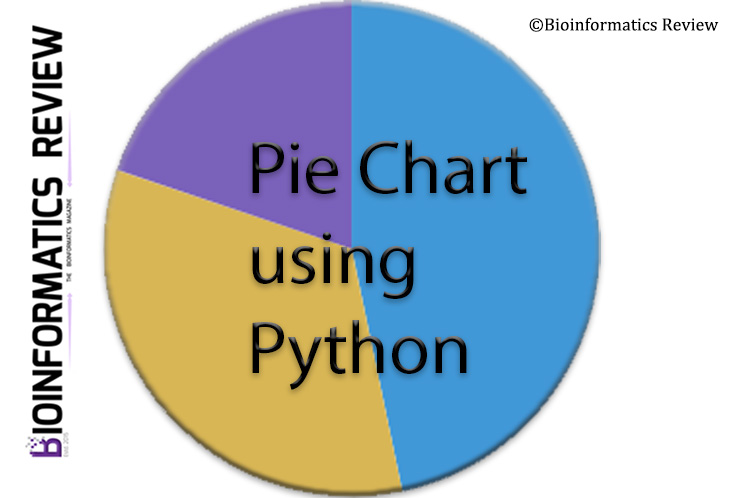
In this article. we are creating a pie chart of the docking score of five different compounds docked with the same protein. (more…)
Bioinformatics Programming
How to make swarm boxplot?
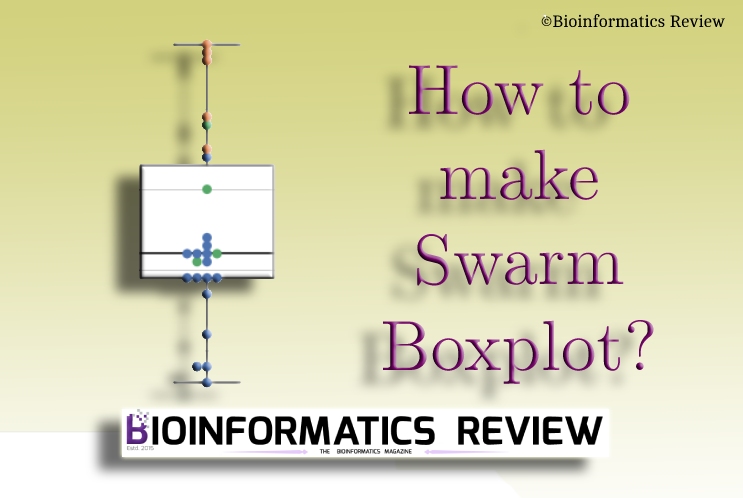
With the new year, we are going to start with a very simple yet complicated topic (for beginners) in bioinformatics. In this tutorial, we provide a simple code to plot swarm boxplot using matplotlib and seaborn. (more…)
Bioinformatics Programming
How to obtain ligand structures in PDB format from PDB ligand IDs?
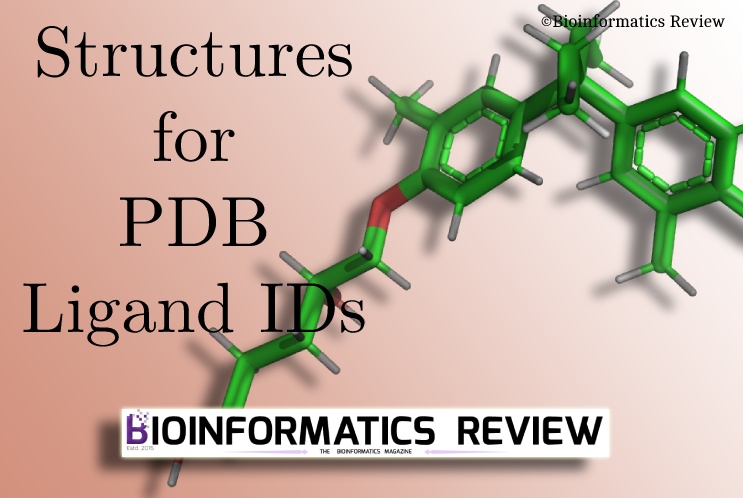
Previously, we provided a similar script to download ligand SMILES from PDB ligand IDs. In this article, we are downloading PDB ligand structures from their corresponding IDs. (more…)
Bioinformatics Programming
How to obtain SMILES of ligands using PDB ligand IDs?
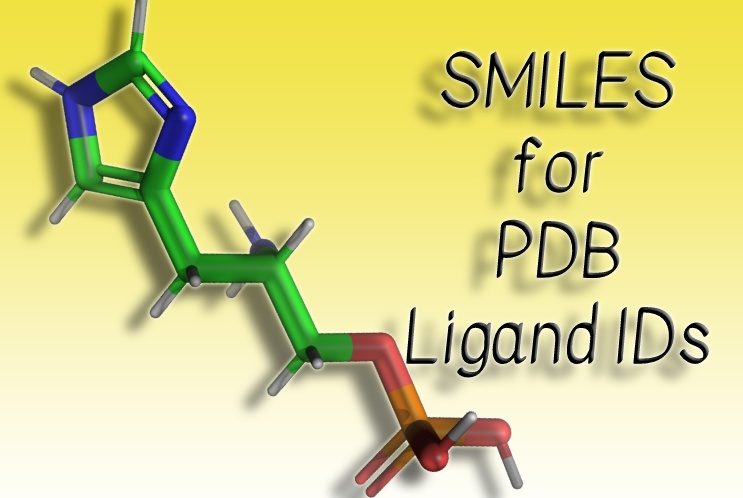
Fetching SMILE strings for a given number of SDF files of chemical compounds is not such a trivial task. We can quickly obtain them using RDKit or OpenBabel. But what if you don’t have SDF files of ligands in the first place? All you have is Ligand IDs from PDB. If they are a few then you can think of downloading SDF files manually but still, it seems time-consuming, especially when you have multiple compounds to work with. Therefore, we provide a Python script that will read all Ligand IDs and fetch their SDF files, and will finally convert them into SMILE strings. (more…)
Bioinformatics Programming
How to get secondary structure of multiple PDB files using DSSP in Python?
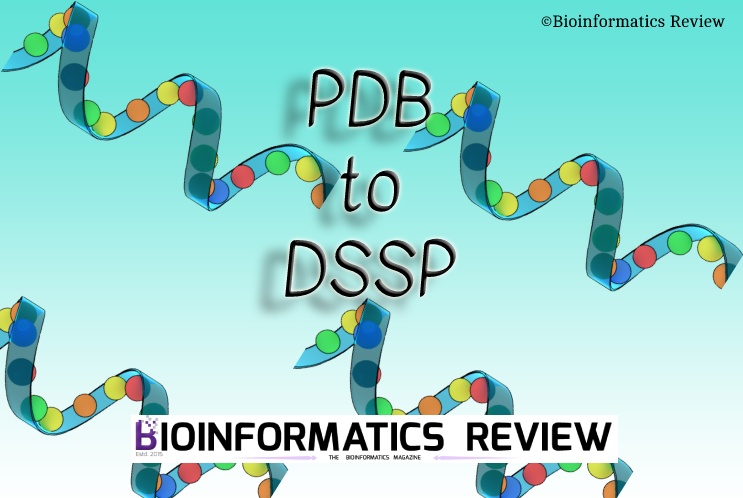
In this article, we will obtain the secondary structure of multiple PDB files present in a directory using DSSP [1]. You need to have DSSP installed on your system. (more…)
Bioinformatics Programming
vs_analysis_compound.py: Python script to search for binding affinities based on compound names.
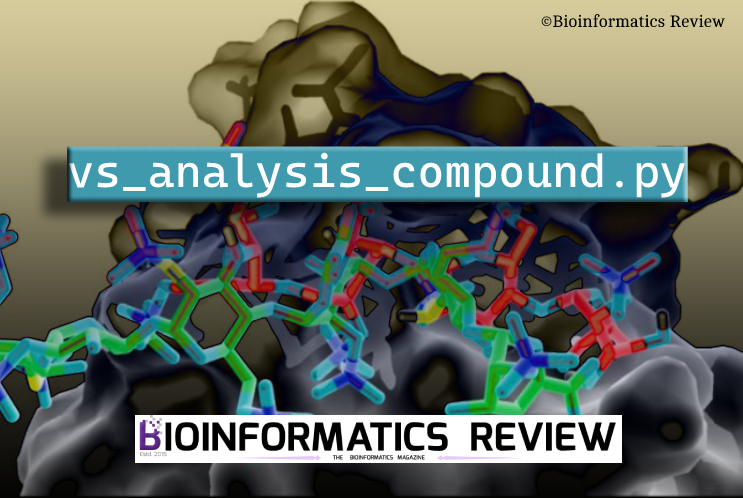
Previously, we have provided the vs_analysis.py script to analyze virtual screening (VS) results obtained from Autodock Vina. In this article, we have provided another script to search for binding affinity associated with a compound. (more…)
Bioinformatics Programming
How to download files from an FTP server using Python?
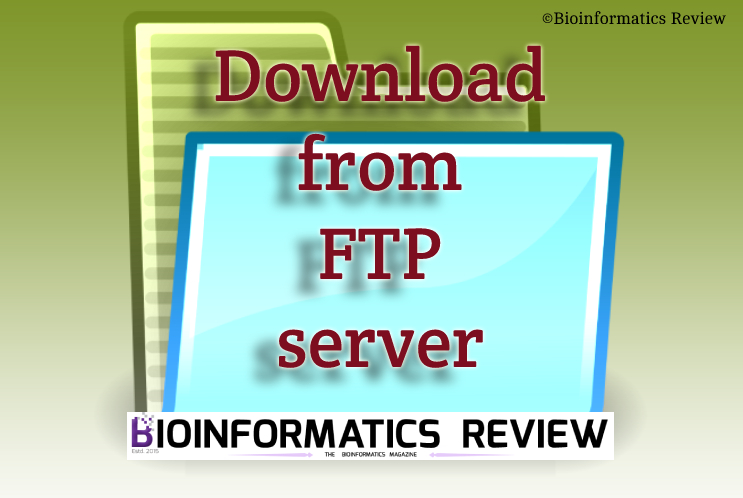
In this article, we provide a simple Python script to download files from an FTP server using Python. (more…)
Bioinformatics Programming
How to convert the PDB file to PSF format?
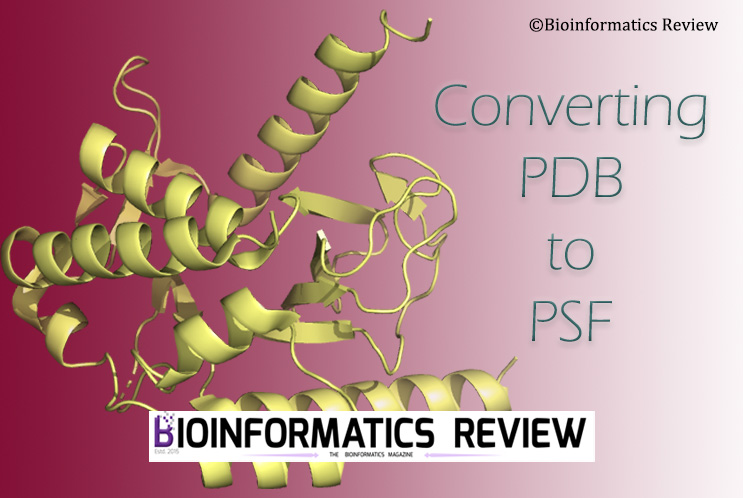
VMD allows converting PDB to PSF format but sometimes it gives multiple errors. Therefore, in this article, we are going to convert PDB into PSF format using a different method. (more…)
Bioinformatics Programming
smitostr.py: Python script to convert SMILES to structures.
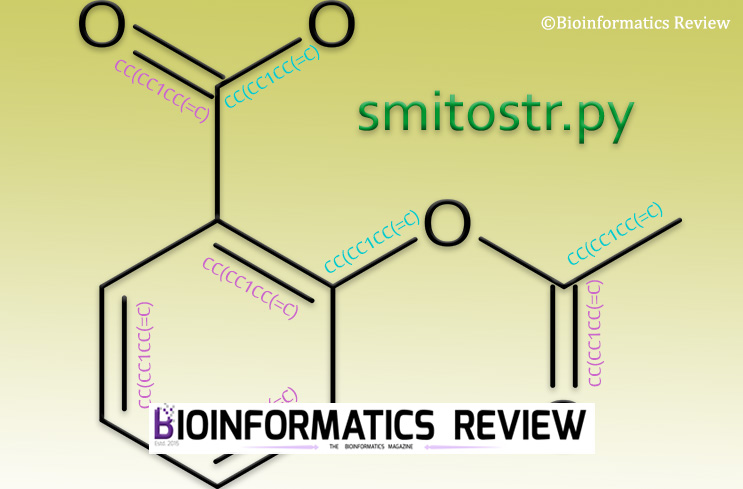
As mentioned in some of our previous articles, RDKit provides a wide range of functions. In this article, we are using RDKit [1] to draw a molecular structure using SMILES. (more…)
Bioinformatics Programming
How to preprocess data for clustering in MATLAB?
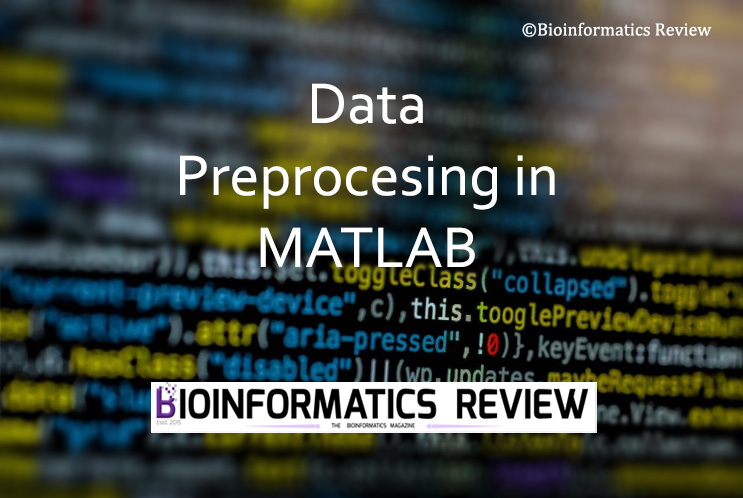
Data preprocessing is a foremost and essential step in clustering based on machine learning methods. It removes noise and provides better results. In this article, we are going to discuss the steps involved in data preprocessing using MATLAB [1]. (more…)
Bioinformatics Programming
How to calculate drug-likeness using RDKit?
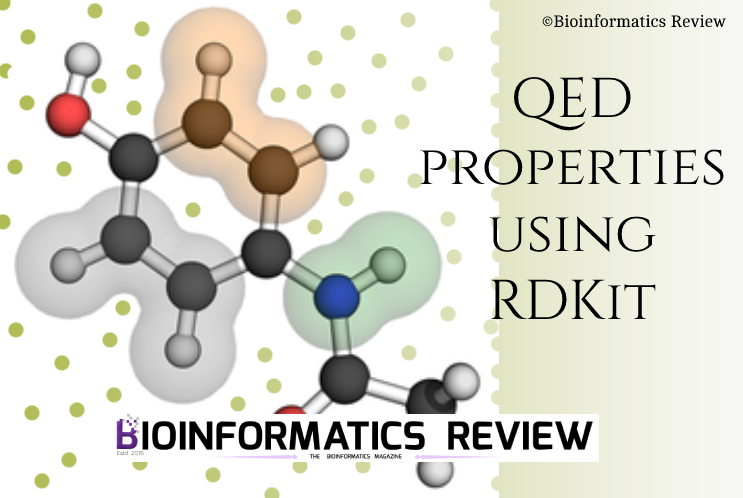
RDKit [1] allows performing multiple functions on chemical compounds. One is the quantitative estimation of drug-likeness also known as QED properties. These properties include molecular weight (MW), octanol-water partition coefficient (ALOGP), number of hydrogen bond donors (HBD), number of hydrogen bond acceptors (HBA), polar surface area (PSA), number of rotatable bonds (ROTB), number of aromatic rings (AROM), structural alerts (ALERTS). (more…)
Bioinformatics Programming
sdftosmi.py: Convert multiple ligands/compounds in SDF format to SMILES.
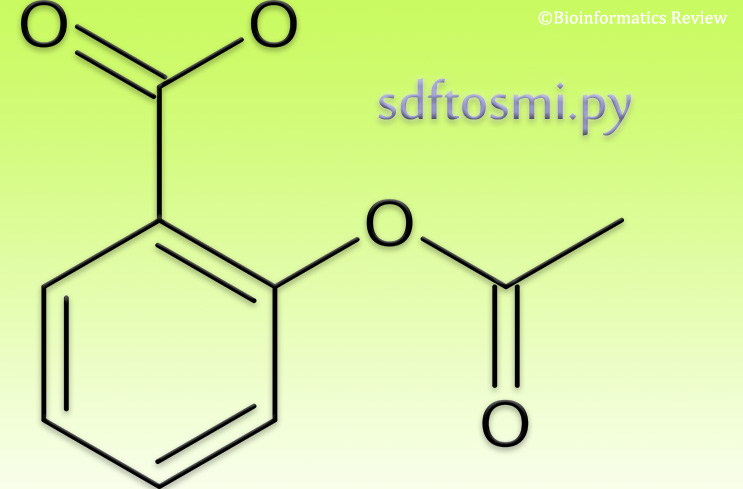
You can obtain SMILES of multiple compounds or ligands in an SDF file in one go. Here, we provide a simple Python script to do that. (more…)
Bioinformatics Programming
tanimoto_similarities_one_vs_all.py – Python script to calculate Tanimoto Similarities of multiple compounds
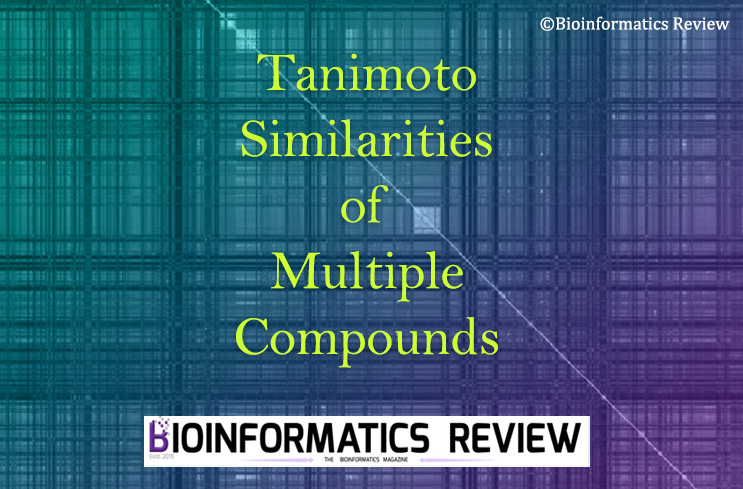
We previously provided a Python script to calculate the Tanimoto similarities of multiple compounds against each other. In this article, we are providing another Python script to calculate the Tanimoto similarities of one compound with multiple compounds. (more…)
Bioinformatics Programming
tanimoto_similarities.py: A Python script to calculate Tanimoto similarities of multiple compounds using RDKit.
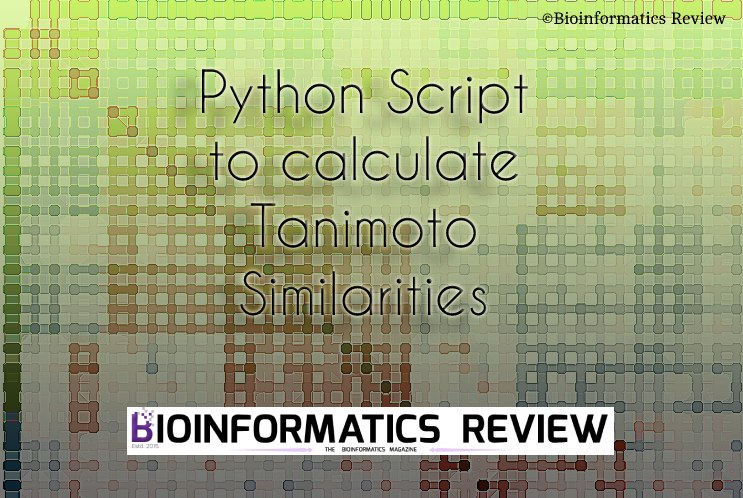
RDKit [1] is a very nice cheminformatics software. It allows us to perform a wide range of operations on chemical compounds/ ligands. We have provided a Python script to perform fingerprinting using Tanimoto similarity on multiple compounds using RDKit. (more…)
Bioinformatics Programming
How to commit changes to GitHub repository using vs code?
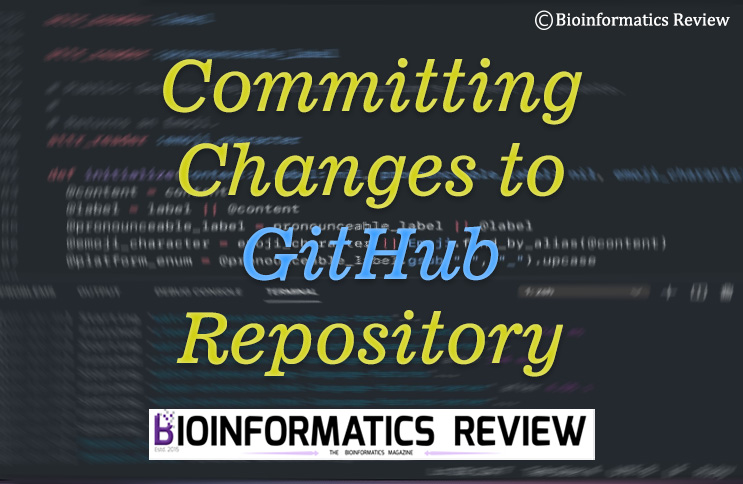
In this article, we are providing a few commands that are used to commit changes to GitHub repositories using VS code terminal.
Bioinformatics Programming
Extracting first and last residue from helix file in DSSP format.
Bioinformatics Programming
How to extract x,y,z coordinates of atoms from PDB file?
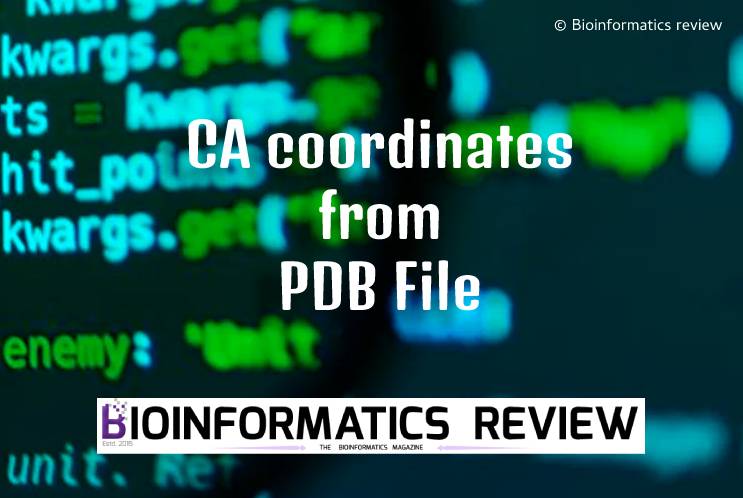
The x, y, and z coordinates of atoms are provided in the PDB file. One way to extract them is by using the Biopython package [1]. In this article, we will extract coordinates of C-alpha atoms for each residue from the PDB file using Biopython. (more…)
Bioinformatics Programming
dssp_parser: A new Python package to extract helices from DSSP files.
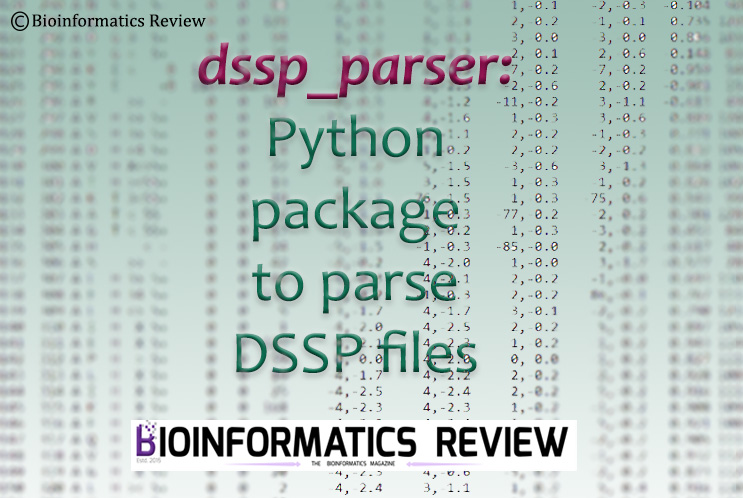
A new Python package named ‘dssp_parser‘ is developed to parse DSSP files. This package fetches all helices including long and short ones from DSSP files. (more…)
Bioinformatics Programming
How to calculate center of mass of a protein structure using Python script?
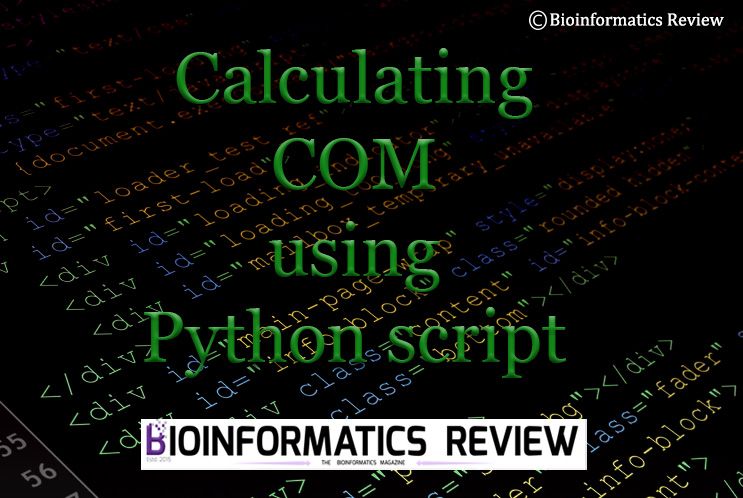
Here is a Python script that helps you calculate the center of mass of a protein using the Pymol [1]. (more…)
Bioinformatics Programming
How to sort binding affinities based on a cutoff using vs_analysis.py script?
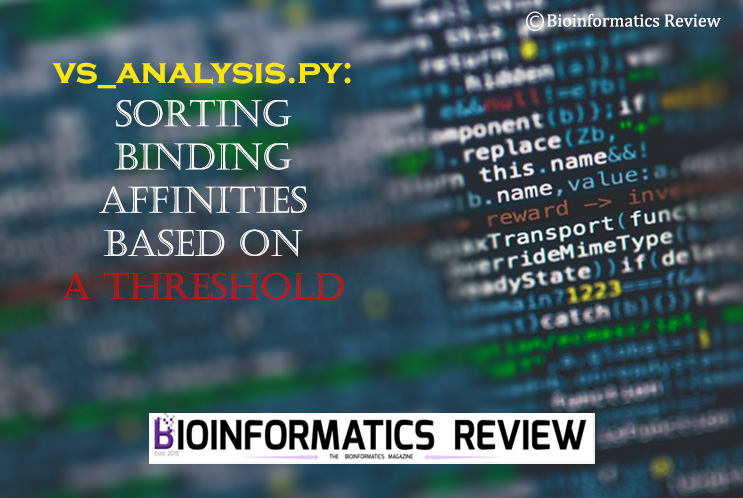
Previously, we have provided a Python script (vs_analysis.py) to analyze the virtual screening (VS) results of Autodock Vina. Now, we have updated this script to sort binding affinities based on user inputted cutoff value. (more…)
You must be logged in to post a comment Login